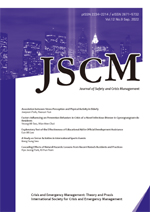
Development of a City-wide Traffic Accident Prediction Model Using Hybrid Machine-based Learning Approaches
Development of a City-wide Traffic Accident Prediction Model Using Hybrid Machine-based Learning Approaches
- 위기관리 이론과 실천
- JSCM(Journal of Safety and Crisis Management)
- Vol.13 No.3
- : KCI등재
- 2023.03
- 49 - 55 (7 pages)
Predicting traffic accidents is a challenging task because taking into account uncertainty in modeling traffic accidents is not trivial. To address these issues, this article develops a hybrid modeling pipeline combining unsupervised and supervised learning to predict the level of hazardous road sites and explore the causality of accidents by controlling unobserved heterogeneity issues effectively. Traffic accident data for Won-ju province, Korea, from 2020 to 2021, and external factors affecting traffic accidents, such as average travel speed and weather information, are combined based on road links. Through the modeling pipeline, a clustering technique is adopted to capture unobserved heterogeneous information among roads. Since traffic accident data contains a wide variety of categorical and hierarchical features, ensemble methods such as boosting techniques were applied to handle heterogeneity issues among these features. To explore the relationship between the accident and determinant factors, are adopted to interpret the results of machine learning models. Model-agnostic methods, however, generally provide results based on images, this study also added a process that extracts texts from images to overcome compatible issues with existing road safety management systems.
Introduction
Data Description
Methodology
Model Analysis
Conclusion
Acknowledgement
References