축약된 Relative Nelson-Siegel 모형과 딥러닝 기법을 이용한 원달러 환율의 변화율 예측
Forecasting KRWUSD Exchange Rate Changes Using a Reduced Relative Nelson-Siegel Model with Deep Learning Techniques
- 한국자료분석학회
- Journal of The Korean Data Analysis Society (JKDAS)
- Vol.25 No.3
-
2023.06987 - 1003 (17 pages)
-
DOI : 10.37727/jkdas.2023.25.3.987
- 32
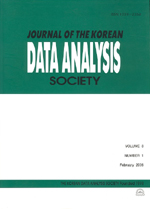
본 연구는 국가 간 이자율 요인(수준, 기울기, 곡도)의 차이에 기반한 Chen, Tsang(2013)의 Relative Nelson-Siegel(RNS) 모형을 원달러 환율 예측에 적용할 때 곡도 또는 곡도와 수준 요인만 사용하는 것이 과대 적합을 피할 수 있음을 보이고(모형 구조의 개선), 비선형성이 고려된 딥러닝 기법이 추가적으로 예측력을 개선하는지(추정 기법의 개선) 검토한다. 실증 분석에서는 2010년 1월부터 2022년 12월까지의 월평균 자료를 이용하여 원달러 환율의 1개월, 3개월, 6개월, 12개월 변화율을 예측하였으며 그 결과는 크게 두 가지로 요약할 수 있다. 첫째, RNS 모형은 과대적합 가능성을 나타낸 반면, 통화정책의 기조 및 미래 단기금리의 (기대수준을 벗어난) 변화를 반영하는 곡도를 중심으로 축약한 RNS 모형은 보다 낮은 RMSE를 나타냈다. 둘째, 1개월 예측의 경우 통계적으로 유의한 모형 간 차이가 발견되지 않았지만 나머지 예측 기간의 경우 딥러닝 기법을 적용한 경우에 예측력이 더욱 개선되었다. 결과적으로 중장기 환율의 변화율을 예측할 때 곡도 요인의 국가 간 차이에 주목하고 추가적인 예측력 개선을 위해 딥러닝 기법을 적극 활용할 필요가 있을 것으로 보인다.
This study applies Chen and Tsang's (2013) Relative Nelson-Siegel (RNS) model, which is based on the differences between the interest rate factors (level, slope, and curvature) of two countries, to forecast the KRWUSD exchange rate and shows that a reduced RNS model with only the curvature or the curvature and level factors can avoid overfitting. Additionally, the study investigates the extent to which deep learning techniques, which consider nonlinearities, can enhance the forecasting performance. The empirical analysis uses monthly average data from January 2010 to December 2022 to forecast the 1-, 3-, 6-, and 12-month exchange rate changes. The main findings are threefold. First, the RNS model tends to suffer from the overfitting, while the reduced RNS model, including a relative curvature factor that reflects cross-country differences in monetary policy stance and unexpected changes in future short-term interest rates, demonstrates a relatively lower RMSE. Second, although no statistically significant model differences were found for the one-month forecast, the forecasting performance for the remaining forecast horizons was improved by deep learning techniques. Therefore, it is necessary to pay attention to cross-country differences in curvature factors when predicting KRWUSD exchange rate changes in the medium to long term and to use deep learning techniques to further improve the forecasting performance.
1. 서론
2. 이론적 배경과 모형 구조
3. 딥러닝 추정 기법
4. 실증분석
5. 결론 및 시사점
References
(0)
(0)