CNN을 활용한 사물 인식 학습 모델 분석
Analyzing an effective object recognition learning model using CNN
- 호서대학교 공업기술연구소
- 공업기술연구 논문집
- 제42권 제1호
-
2023.0633 - 41 (9 pages)
- 86
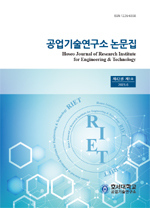
컴퓨터 하드웨어와 비선형문제를 풀 수 있는 기법이 발달하면서 인공지능에 관련된 연구가 활발해지고 특히 영상처리 분야에 다양한 연구가 이루어지고 있다. 본 논문에서는 영상처리에 많이 이용되는 CNN (convolution neural network)를 활용하여 사물 인식 학습 모델을 구성하고, CNN 모델별 성능과 최적화 기법별 성능을 비교하였다. 학습 과정에서 데이터증분(data augmentation)과 K-Fold 교차 검증을 활용하였으며, 최적화기로 SGD, RMSprop, Adam, Nadam를 사용하였다. CNN 모델로 VGG16, VGG19, ResNet50, ResNet152를 사용하였다. 수치적 실험 결과, 최적화기는 SGD를 사용하였을 때 손실 함수값이 빠르게 감소하고, 테스트 데이터 검출률도 가장 높게 나왔다. CNN 모델로는 ResNet50을 사용하였을 때 검출률이 가장 높게 나왔다. 따라서 본 연구에서 대상으로 한 사물 인식의 경우 SGD와 ResNet50이 다른 옵티마이저와 CNN 모델에 비해 성능이 높은 것을 확인할 수 있었다.
With the development of computer hardware and techniques for solving nonlinear problems, research related to artificial intelligence is becoming active, and various studies are being conducted, especially in the field of image processing. In this paper, an object recognition learning model is constructed using convolution neural network(CNN), which is widely used for image processing, and performance by CNN model and performance by optimization technique are compared. In the learning process, data augmentation and K-Fold cross-validation were used, and SGD, RMSprop, Adam, Nadam were used as optimizers. As CNN models, VGG16, VGG19, ResNet50, and ResNet152 were used. As a result of numerical experiments, when the optimizer used SGD, the loss function value decreased rapidly and the test data detection rate was the highest. As a CNN model, the detection rate was the highest when ResNet50 was used. Therefore, it was confirmed that SGD and ResNet50 performed better than other optimizer and CNN models for object recognition in this study.
Ⅰ. 서 론
Ⅱ. 본 론
Ⅲ. 실 험
Ⅳ. 결 론
참고문헌
(0)
(0)