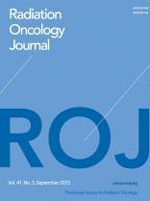
Developing prompts from large language model for extracting clinical information from pathology and ultrasound reports in breast cancer
Developing prompts from large language model for extracting clinical information from pathology and ultrasound reports in breast cancer
- 대한방사선종양학회
- 대한방사선종양학회지
- 제41권 제3호
- : SCOPUS
- 2023.09
- 209 - 216 (8 pages)
Purpose: We aimed to evaluate the time and cost of developing prompts using large language model (LLM), tailored to extract clinical factors in breast cancer patients and their accuracy. Materials and Methods: We collected data from reports of surgical pathology and ultrasound from breast cancer patients who underwent radiotherapy from 2020 to 2022. We extracted the information using the Generative Pre-trained Transformer (GPT) for Sheets and Docs extension plugin and termed this the “LLM” method. The time and cost of developing the prompts with LLM methods were assessed and compared with those spent on collecting information with “full manual” and “LLM-assisted manual” methods. To assess accuracy, 340 patients were randomly selected, and the extracted information by LLM method were compared with those collected by “full manual” method. Results: Data from 2,931 patients were collected. We developed 12 prompts for Extract function and 12 for Format function to extract and standardize the information. The overall accuracy was 87.7%. For lymphovascular invasion, it was 98.2%. Developing and processing the prompts took 3.5 hours and 15 minutes, respectively. Utilizing the ChatGPT application programming interface cost US $65.8 and when factoring in the estimated wage, the total cost was US $95.4. In an estimated comparison, “LLM-assisted manual” and “LLM” methods were time- and cost-efficient compared to the “full manual” method. Conclusion: Developing and facilitating prompts for LLM to derive clinical factors was efficient to extract crucial information from huge medical records. This study demonstrated the potential of the application of natural language processing using LLM model in breast cancer patients. Prompts from the current study can be re-used for other research to collect clinical information.
Introduction
Materials and Methods
Results
Discussion and Conclusion
Statement of Ethics
Conflict of Interest
Funding
Author Contributions
Data Availability Statement
Supplementary Materials
References