동형암호를 활용한 앙상블학습방법의 추론
Inference of Ensemble Method Using Homomorphic Encryption
- 한국자료분석학회
- Journal of The Korean Data Analysis Society (JKDAS)
- Vol.25 No.5
-
2023.101725 - 1734 (10 pages)
-
DOI : 10.37727/jkdas.2023.25.5.1725
- 86
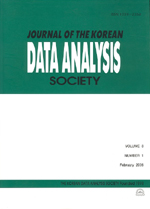
데이터와 관련된 법안이 최근 개정됨에 따라 초 개인화 서비스를 위한 빅데이터 분석이 활발히 이루어지고 있다. 초 개인화 서비스는 대규모 데이터 통합, 외부 데이터와의 결합 등의 기술을 필요로 하지만, 동시에 민감한 개인정보 유출로 인한 개인 및 기업의 피해와 이로 인한 불필요한 사회적 손실에 대한 우려로 인한 사용상의 한계성도 내재하고 있다. 이와 같은 보안위험을 줄이기 위한 여러 방안 중 개인정보가 보호되는 동형암호(homomorphic encryption) 기술을 고려할 수 있다. 특히, 동형암호는 데이터 결합과 분석과정에서 개인정보 유출을 원천적으로 차단함과 더불어 원본 데이터의 정보를 보존할 수 있는 특징을 가지고 있다. 본 연구에서는 실제 금융 분야의 민원자료에 동형암호를 활용한 앙상블학습방법(ensemble method)의 추론을 수행하는 알고리즘을 개발 및 적용하여 통상적인 평문(plaintext)에서 도출한 random forest와 XGBoost의 스코어와 암호문(ciphertext)에서의 두 방법을 통해 산출한 스코어의 값이 일치함을 보이고자 한다. 실증결과로, 약 0.4초의 단위 추론 계산시간을 달성하여 트리구조에 기반한 앙상블학습방법의 활용 가능성을 확인할 수 있었다.
According to the recent amendment of data-related bills, active efforts are being made to conduct big data analysis for hyper-personalization services. Hyper-personalization services involve several data integration, including external data. However, it also inherently possesses limitations in usage due to concerns about private information leaks, which can lead to damages to individuals and businesses and unnecessary societal losses. One approach to mitigate such security risks is to use homomorphic encryption, which protects private information. In particular, homomorphic encryption fundamentally prevents private information leakage during data integration and analysis processes and retains original data. In this study, we develop an algorithm for performing inference using an ensemble learning method with homomorphic encryption and apply it to real financial complaint data. We demonstrate the equivalence between scores obtained from random forest and XGBoost in plaintext and those derived from the homomorphic ciphertext. Empirical results show that the algorithm achieves inference computation times in approximately 0.4 seconds, confirming the potential utility of the tree-based ensemble learning method.
1. 서론
2. 분석방법론
3. 실제 자료 분석
4. 결론
References
(0)
(0)