XAI SHAP 기반 토지 피복 구성에 따른 지표면 온도의 영향 분석
Analysis of the Effect of Surface Temperature in accordance with the Composition of Land Cover Based on XAI SHAP
- 한국자료분석학회
- Journal of The Korean Data Analysis Society (JKDAS)
- Vol.25 No.5
-
2023.101735 - 1748 (14 pages)
-
DOI : 10.37727/jkdas.2023.25.5.1735
- 27
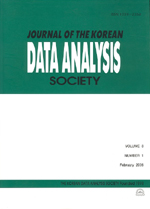
최근 인공지능 아키텍처의 발전과 하드웨어의 성능이 급속도로 개선되면서 다양한 인공지능모델들의 유용성이 증가하고 있다. 갈수록 복잡해지고 있는 인공지능 모델의 의사결정 분기점은 기하급수적으로 늘어나고 있으며, 이는 연구자의 모델 해석 용이성을 저해시키는 원인이 된다. 이에 따라 블랙박스 모형으로 알려진 인공지능 모형을 설명하기 위한 연구도 빠르게 발전하고 있다. 설명 가능한 인공지능(eXplainable Artificial Intelligence, XAI)은 위와 같은 복잡성을 해소하기 위해 등장하였으며, 모델의 블랙박스를 연구자가 이해 가능한 수준으로 분해하여 해석 가능성 및 신뢰도 제고에 도움을 준다. 본 연구는 서울특별시 셀(cell) 단위의 토지 피복 데이터를 이용하여 특정 공간 단위의 지표면 온도를 추정한다. 고차원 데이터에 대한 기존 통계모형의 한계를 살펴보았으며, 머신러닝 모델을 이용하여 추정 결과를 비교하였다. 사용한 모형으로는 Lasso(Least absolute shrinkage and selection operator) 회귀, 랜덤포레스트 회귀(random forest regression), XGBoost(eXtreme Gradient Boosting)가 있으며, XGBoost 모델의 적합 결과를 바탕으로 SHAP(SHapley Additive exPlanation)을 통한 XAI 점검을 시행하였다.
Recently, with the development of artificial intelligence architecture and the rapid improvement of hardware performance, various artificial intelligence models are attracting attention and their usefulness are increasing. However, unlike the rate of development of performance, the development of model's explanatory ability is slow to progress. The increasingly complex decision branching points of AI models are increasing exponentially, which hinders researchers' ease of model interpretation. Explainable Artificial Intelligence, XAI has emerged to solve the above complexity, and helps to improve interpretability and reliability by decomposing the black box of the model to a level that researchers can understand. This study estimates the surface temperature of a specific spatial unit using land cover data of a cell unit in Korea, Seoul. The limitations of the existing statistical model for high-dimensional data were examined, and the estimation results were compared using a machine learning model. Models used include Lasso regression(Least absolute linkage and selection operator), random forest regression, and XGBoost (eXtreme Gradient Boosting) regression. Finally, based on the fitted result of the XGBoost regression model, XAI SHAP was carried out.
1. 서론
2. 지표면 온도 및 설명자료 측정 데이터
3. 선형회귀모형 및 머신러닝 모델을 이용한 지표면 온도 추정
4. XAI SHAP 기반 모델의 점검
5. 결론
References
(0)
(0)