범주 불균형 금융 문제의 해결을 위한 부스팅 학습
Boosting for Addressing Class Imbalance Problems in Financ
- 한국자료분석학회
- Journal of The Korean Data Analysis Society (JKDAS)
- Vol.25 No.5
-
2023.101859 - 1873 (15 pages)
-
DOI : 10.37727/jkdas.2023.25.5.1859
- 37
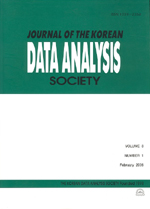
범주 불균형 문제는 분류 및 예측 모형의 성과 개선을 위하여 필수적으로 해결해야 할 문제이다. 본 연구는 금융 분야에서 나타나는 범주 불균형 문제를 해결하기 위해 AUC 성과 지표에 대한 직접적인 최적화를 도입한 AUCBoost 기법을 제안한다. 본 연구에서는 데이터의 불균형 정도에 따른 알고리즘의 효과를 비교하고자 기업 부도, 카드 사기, 카드 연체를 대상으로 AUCBoost의 성과를 검증하였다. 본 연구는 성과 검증을 위하여 로지스틱 통계 모형과 AdaBoost, GBM, XGBoost 부스팅 알고리즘을 벤치마킹 모형으로 채택하였으며 10-fold 교차 타당성 검증을 3회 반복하였다. 주요 분석 결과는 다음과 같다. 첫째, 다수 범주에 초점을 맞추는 벤치마킹 모형과 비교하여 AUCBoost는 다수 범주와 소수 범주를 동시에 고려하는 균형 학습으로 범주 불균형 문제에 대하여 AUC 측면에서 유의한 성과 개선 효과를 보여주었다. 둘째, 범주 불균형 데이터에 대한 반복측정 분산분석 결과 AUCBoost는 벤치마킹 모형과 비교하여 AUC 측면에서 유의한 성과 차이를 보여주었다. 셋째, 데이터 샘플링을 적용한 범주 균형 데이터에서도 벤치마킹 모형과 비교하여 AUCBoost는 AUC 측면에서 유의한 성과 개선 효과를 보여주었다.
The class imbalance problem is essential to be solved in order to improve the performance of classification and prediction models. This study proposes an AUCBooost technique that introduces a direct performance optimization technique to improve the performance of boosting algorithms applied to class imbalance problems in the financial sector. In this study, the performance of AUCBoost is verified on class imbalance problems such as corporate bankruptcy, card insolvency, and card fraud. For comparative analysis of performance, logistic, AdaBoost, GBM, and XGBoost are adopted as benchmark models. The results of repeated 10-fold cross-validation are as follows. First, unlike conventional algorithms only focusing on majority class in class imbalance problems, AUCBoost shows a balanced learning behavior that simultaneously considers the specificity of multiple class and the sensitivity of minority class. Second, compared to the benchmarking model, RMA shows that AUCBoost shows significantly improved performance in terms of AUC. Third, in class balanced data generated from data sampling, AUCBooost also shows the significantly improved performance compared to benchmarking models.
1. 서론
2. 이론적 배경 및 가설설정
3. 제안 알고리즘: AUCBoost
4. 자료 및 모형설계
5. 연구결과
6. 결론
References
(0)
(0)