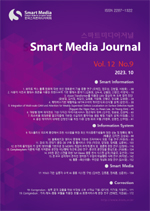
익스플리싯 피드백 환경에서 추천 시스템을 위한 최신 지식증류기법들에 대한 성능 및 정확도 평가
State-of-the-Art Knowledge Distillation for Recommender Systems in Explicit Feedback Settings: Methods and Evaluation
- 한국스마트미디어학회
- 스마트미디어저널
- Vol12, No.9
- 2023.10
- 89 - 94 (6 pages)
추천 시스템은 사용자가 아이템에 남긴 익스플리싯 또는 임플리싯 피드백을 바탕으로 각 사용자가 선호할 법한 아이템들을 추천하는 기술이다. 최근, 추천 시스템에 사용되는 딥 러닝 기반 모델의 사이즈가 커짐에 따라, 높은 추천 정확도를 유지하며 추론 시간은 줄이기 위한 목적의 연구가 활발히 진행되고 있다. 대표적으로 지식증류기법을 이용한 추천 시스템에 관한 연구가 있으며, 지식증류기법이란 큰 사이즈의 모델(즉, 교사)로부터 추출된 지식을 통해 작은 사이즈의 모델(즉, 학생)을 학습시킨 뒤, 학습이 끝난 작은 사이즈의 모델을 추천 모델로서 이용하는 방법이다. 추천 시스템을 위한 지식증류기법들에 관한 기존의 연구들은 주로 임플리싯 피드백 환경만을 대상으로 수행되어 왔었으며, 본 논문에서 우리는 이들을 익스플리싯 피드백 환경에 적용할 경우의 성능 및 정확도를 관찰하고자 한다. 실험을 위해 우리는 총 5개의 최신 지식증류기법들과 3개의 실세계 데이터셋을 사용하였다.
Recommender systems provide users with the most favorable items by analyzing explicit or implicit feedback of users on items. Recently, as the size of deep-learning-based models employed in recommender systems has increased, many studies have focused on reducing inference time while maintaining high recommendation accuracy. As one of them, a study on recommender systems with a knowledge distillation (KD) technique is actively conducted. By KD, a small-sized model (i.e., student) is trained through knowledge extracted from a large-sized model (i.e., teacher), and then the trained student is used as a recommendation model. Existing studies on KD for recommender systems have been mainly performed only for implicit feedback settings. Thus, in this paper, we try to investigate the performance and accuracy when applied to explicit feedback settings. To this end, we leveraged a total of five state-of-the-art KD methods and three real-world datasets for recommender systems.
Ⅰ. 서론
Ⅱ. 본론
Ⅲ. 결론
REFERENCES