넙치 질병 증상 분류를 위한 객체 탐지 딥러닝 모델 성능 평가
Performance Evaluation of Object Detection Deep Learning Model for Paralichthys olivaceus Disease Symptoms Classification
- 한국스마트미디어학회
- 스마트미디어저널
- Vol12, No.10
-
2023.1171 - 84 (14 pages)
-
DOI : 10.30693/SMJ.2023.12.10.71
- 51
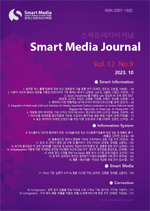
넙치 양식은 우리나라 양식 산업의 절반 이상 차지할 정도로 큰 비중을 차지한다. 그러나 연중 총사육량의 25~30% 정도가 질병으로 인한 집단 폐사가 발생하여 양식장의 경제성에 매우 나쁜 영향을 준다. 넙치 양식장의 경제성 성장을 위해서는 넙치 질병 증상 진단을 자동화하여 빠르고 정확하게 질병 진단을 하는 방법이 필요하다. 본 연구에서는 독창적인 학습 데이터 수집 방법과 학습 정제 알고리즘 및 학습 데이터 분리 기법을 사용하여 학습 데이터를 구축하고 4가지 객체 탐지 딥러닝 모델(YOLOv8, Swin, Vitdet, MvitV2)의 넙치 질병 증상 감지 성능을 비교한다. 실험 결과 YOLOv8 모델이 평균 인식률(mAP)과 예상 도착 시간(ETA) 관점에서 우수하다는 결론을 얻었다. 본 연구에서 제안하는 AI 모델의 성능이 검증되면 넙치 양식장에서는 실시간으로 넙치 질병을 진단할 수 있고, 진단 결과에 따른 신속한 예방 조치로 양식장의 생산성은 크게 향상될 것이라 기대된다.
Paralichthys olivaceus accounts for a large proportion, accounting for more than half of Korea's aquaculture industry. However, about 25-30% of the total breeding volume throughout the year occurs due to diseases, which has a very bad impact on the economic feasibility of fish farms. For the economic growth of Paralichthys olivaceus farms, it is necessary to quickly and accurately diagnose disease symptoms by automating the diagnosis of Paralichthys olivaceus diseases. In this study, we create training data using innovative data collection methods, refining data algorithms, and techniques for partitioning dataset, and compare the Paralichthys olivaceus disease symptom detection performance of four object detection deep learning models(such as YOLOv8, Swin, Vitdet, MvitV2). The experimental findings indicate that the YOLOv8 model demonstrates superiority in terms of average detection rate (mAP) and Estimated Time of Arrival (ETA). If the performance of the AI model proposed in this study is verified, Paralichthys olivaceus farms can diagnose disease symptoms in real time, and it is expected that the productivity of the farm will be greatly improved by rapid preventive measures according to the diagnosis results
Ⅰ. 서론
Ⅱ. 관련 연구
Ⅲ. 넙치 질병 증상 감지를 위한 학습 데이터 구축 및 정제 기법
Ⅳ. 넙치 질병 감지를 위한 객체 탐지모델 학습 및 성능 평가
Ⅴ. 결론
REFERENCE
(0)
(0)