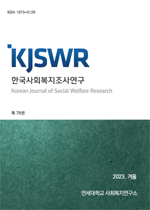
사회복지의 상담기록, 좀 더 활용할 수 있을까? ‘머신러닝’을 통한 사회복지 상담 텍스트 활용 가능성의 점검
To understand machine-learning text analysis in order to more utilize interview text in the area of social welfare
- 연세대학교 사회복지연구소
- 한국사회복지조사연구
- 79권
- 2023.12
- 5 - 26 (22 pages)
사회복지 영역은 다양한 내용과 형태의 텍스트 데이터를 생산 및 보유하고 있지만, 비정형 데이터가 활발히 분석되고 있는 시대에 비추어 볼 때, 이의 분석과 활용에는 아쉬움이 있다. 본 연구는여기에 머신러닝(혹은 인공지능) 방법에 대한 거리감도 작용하고 있는 것으로 보고, 이 방법의원리를 이해할 수 있도록 과정을 찬찬히 살펴보고, 한계와 가능성을 함께 생각해 보고자 하였다. 이를 위해 소규모 실험용 데이터 세트를 구축하고, 설문조사 자료에 기반한 추론통계 판별 모델과텍스트 데이터에 기반한 머신러닝 예측 모델을 비교하며 검토하였다. 텍스트 데이터의 분석은 비정형의 텍스트를 수치 표현으로 바꿔주는 과정을 거치며, 이는 놀랄만큼 많은 양의 변수(특성)를 다루는 것을 의미하지만, 개념적으로 새로울 것은 없었다. 예측 모델구축 방법도 이항 로지스틱 회귀분석 등의 익숙한 방법을 주로 이용하지만, 모집단 추론 가능성이아니라 예측 성능으로 모델을 평가하므로, 훈련 데이터와 테스트 데이터의 엄격한 분리, 모델의훈련, 모델의 테스트로 이어지는 과정이 달랐다. 텍스트 데이터에 기초한 머신러닝 예측 모델의우수한 예측력은 부분적으로는 여러 ‘잡음’까지도 포함된 방대한 양의 정보를 분석하기 때문인것으로 보이며, 그만큼 해석이 쉽지 않고, 학습 데이터의 주기적 업데이트와 리모델링이 요구됨을알 수 있었다. 이러한 머신러닝 모델의 원리와 한계의 이해에 기초하여 사회복지 상담 텍스트의활용과 관련한 다양한 가능성이 상상될 수 있을 것으로 보인다.
This study aims to examine the machine learning analysis of text data step by step in order to enable colleagues in the field to conceptually understand the method and to think about the limit and possibility of it. Analyzing text data requires the process of transform atypical text data into numerical expression, which means we need to deal with surprisingly large amount of variables (that is, features), but at the same time, means there is no ‘magical’ thing that we cannot understand. The process of constructing a prediction model also uses methods familiar to us such as binary logistics regression, although those are not all of the list of methods. However, machine learning model is evaluated not by inferential validity but by predicting ability, which shows approaches of Big data analysis and of conventional inferential statistical analysis are fundamentally different. Thus, machine learning model requires the processes of strictly dividing training data and test data, of training, and of test. It appears the impressive performance of machine learning prediction model due to its ability to analyze a great amount of information encompassing even ‘noise’-looking ones. Therefore, it is not easy and straightforward to interpret the result, and it might be important to update training data and re-model frequently.
I. 문제제기와 연구 필요성
II. 데이터와 연구 방법
III. 분석결과
IV. 결론
참고문헌