Seq2Seq 모델을 활용한 교육용 챗봇 구현에 관한 연구
A Study on the Implementation of Educational Chatbot Using Seq2Seq Model
- 한국산업기술융합학회(구. 산업기술교육훈련학회)
- 산업기술연구논문지
- 제28권 4호
-
2023.12135 - 143 (9 pages)
-
DOI : 10.29279/jitr.2023.28.4.135
- 46
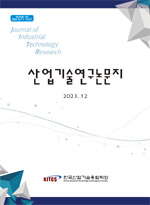
모바일 기기의 발전과 메신저 사용의 활성화로 학생들과 소통하는 방법으로 챗봇 사용의 필요성이 증대되고 있다. 학생들 간의 수준 차이로 인해 개인들이 다양한 질문을 하고, 맞춤형 답변을 받을 수 있다면 학업 성취도 향상에도움이 될 것이다. 학생들이 챗봇과 자연스럽게 대화하기 위해서 조지아텍의 질 왓슨처럼 친숙하고 정확한 답변을 제공할 수 있어야 한다. 본 논문에서는 자연어 처리가 가능하고, LSTM 구조의 Seq2Seq 알고리즘을 개선한 챗봇을 구현하였다. 구현된 챗봇은 일상생활 데이터를 가지고 학습하였고 빠른 응답성과 높은 정확도, 낮은 손실율을 보였고답변 내용도 우수하였다.
With the development of mobile devices and the activation of messenger use, it is necessary to use chatbot as a communication tool with students. If individuals can ask various questions and receive customized answers owing to differences in levels among students, it will help improve academic achievement. For students to naturally communicate with chatbot, they must be able to provide familiar and accurate answers similar to those of Georgia Tech's Jill Watson. In this study, a chatbot capable of natural language processing and improving the Seq2Seq algorithm of the long short-term memory structure was implemented. The implemented chatbot learned from the open daily life conversation data, and consequently, it exhibited high accuracy and low loss rate within a short time, and the answer was excellent.
Ⅰ. 서 론
Ⅱ. 챗봇과 자연어 처리
Ⅲ. 딥러닝 모델과 알고리즘
Ⅳ. 학습용 챗봇 모델
Ⅴ. 결 론
참고문헌
(0)
(0)