앙상블 머신러닝 모델을 활용한 치매 예측
Predicting Dementia Using Ensemble Machine Learning Models: Focusing on Exercise and Sleep Information
- 제주대학교 관광과경영경제연구소
- 산경논집
- Vol. 43 No. 2
-
2023.1291 - 109 (19 pages)
-
DOI : 10.24907/JTIR.2023.43.2.91.
- 205
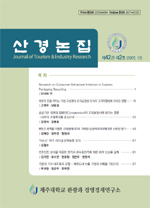
고령화 사회가 되어가면서 노인 질병에 관해 관심이 높아지고 있다. 그 중, 일상생활에 지장을 많이 주는 노인성 치매는 기술이 발달하여도 완치할 수 있는 치료법이 없기 때문에 초기에 발견하는 것이 가장 좋은 방법이다. 하지만 이러한 치매 예측을 위하여 수많은 사전 연구들에서는 MRI, 망막 이미지 영상 데이터 등 지속적인 촬영과 비용이 필요하다. 이러한 방법들은 모든 사람들에게 적용시킬 수 없다는 단점을 가지고 있다. 이에 본 연구에서는 치매 예측 발견의 저비용에 관한 발전을 위하여 운동 시간, 고강도 활동 시간, 신진대사량 등 여러 활동 데이터와 깊은 수면 시간, 분 당 호흡 수 등 수면의 질을 알 수 있는 수면 데이터를 활용하여 XGBsoot 와 lightGBM과 같은 딥러닝 예측 모델을 이용하여 치매 여부에 가장 영향을 많이 미친 주요 변수들을 알아내는 것을 목적으로 한다. 분석 결과, 활동 데이터와 수면 데이터를 동시에 고려했을 때 치매 예측 성능이 가장 잘 나왔고, XGBoost 모델에서 가장 중요한 변수는 ‘매 시간당 활동 유지 점수’이었고, lightGBM은 ‘분당 평균 심박동 수’ 이었다.
As we become an aging society, there is a growing concern about geriatric diseases. Among them, senile dementia, which disrupts daily life, is best detected at an early stage because there is no cure, even with technological advances. However, many preliminary studies to predict dementia require continuous imaging data, such as MRI and retinal imaging data, and are expensive. These methods have the disadvantage that they cannot be applied to all people, so this study aims to develop low-cost predictive dementia detection. Therefore, the purpose of this study is to identify the main variables that most influenced the presence of dementia using deep learning prediction models such as XGBsoot and lightGBM by utilizing various activity data such as exercise duration, high-intensity activity duration, and metabolic rate, and sleep data such as deep sleep duration and breathing rate per minute to determine sleep quality. The analysis showed that the best dementia prediction performance was achieved when both activity and sleep data were considered simultaneously, and the most important variable in the XGBoost model was the “activity_score_move_every_hour” and the “sleep_hr_everage” in lightGBM.
Ⅰ. 서 론
Ⅱ. 관련 연구
Ⅲ. 데이터 수집 및 전처리(신명조, 13)
Ⅳ. 데이터 분석
Ⅴ. 결과
Ⅵ. 결론
참고문헌
(0)
(0)