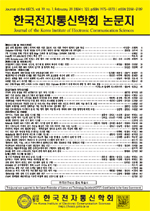
SAC 강화 학습을 통한 스마트 그리드 효율성 향상
Enhancing Smart Grid Efficiency through SAC Reinforcement Learning: Renewable Energy Integration and Optimal Demand Response in the CityLearn Environment
- 한국전자통신학회
- 한국전자통신학회 논문지
- 제19권 제1호
- 2024.02
- 93 - 104 (12 pages)
수요 반응은 전력망의 신뢰성을 높이고 비용을 최소화하기 위해 수요가 가장 많은 시간대에 고객이 소비 패턴을 조정하도록 유도한다. 재생 에너지원을 스마트 그리드에 통합하는 것은 간헐적이고 예측할 수 없는 특성으로 인해 상당한 도전 과제를 안고 있다. 강화 학습 기법과 결합된 수요 대응 전략은 이러한 문제를 해결하고 기존 방식에서는 이러한 종류의 복잡한 요구 사항을 충족하지 못하는 경우 그리드 운영을 최적화할 수 있는 접근 방식으로 부상하고 있다. 본 연구는 재생 에너지 통합을 위한 수요 반응에 강화 학습 알고리즘을 적용하는 방법을 찾아 적용하는데 중점을 둔다. 연구의 핵심 목표는 수요 측 유연성을 최적화하고 재생 에너지 활용도를 개선할 뿐 아니라 그리드 안정성을 강화하고자 한다. 연구 결과는 강화 학습을 기반으로 한 수요 반응 전략이 그리드 유연성을 향상시키고 재생 에너지 통합을 촉진하는 데 효과적이라것을 보여준다.
Demand response is a strategy that encourages customers to adjust their consumption patterns at times of peak demand with the aim to improve the reliability of the power grid and minimize expenses. The integration of renewable energy sources into smart grids poses significant challenges due to their intermittent and unpredictable nature. Demand response strategies, coupled with reinforcement learning techniques, have emerged as promising approaches to address these challenges and optimize grid operations where traditional methods fail to meet such kind of complex requirements. This research focuses on investigating the application of reinforcement learning algorithms in demand response for renewable energy integration. The objectives include optimizing demand-side flexibility, improving renewable energy utilization, and enhancing grid stability. The results emphasize the effectiveness of demand response strategies based on reinforcement learning in enhancing grid flexibility and facilitating the integration of renewable energy.
Ⅰ. Introduction
Ⅱ. Methodology
Ⅲ. Experiments and results
Ⅳ. Key findings
Ⅴ. Discussion
Ⅵ. Conclusion
References