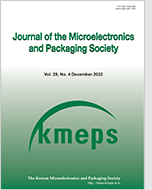
MAGICal Synthesis
MAGICal Synthesis: Memory-Efficient Approach for Generative Semiconductor Package Image Construction
- 한국마이크로전자및패키징학회
- 마이크로전자 및 패키징학회지
- 제30권 제4호
- 2023.12
- 69 - 78 (10 pages)
산업 인공지능의 발달과 함께 반도체의 수요가 크게 증가하고 있다. 시장 수요에 대응하기 위해 패키징 공정에서 자동 결함 검출의 중요성 역시 증가하고 있다. 이에 따라, 패키지의 자동 불량 검사를 위한 딥러닝 기반의 방법론들의 연구가 활발히 이루어 지고 있다. 딥러닝 기반의 모델은 학습을 위해서 대량의 고해상도 데이터를 필요로 하나, 보안이 중요한 반도체 분야의 특성상 관련 데이터의 공유 및 레이블링이 쉽지 않아 모델의 학습이 어려운 한계를 지니고 있다. 또한 고해상도 이미지를 생성하기 위해 상당한 컴퓨팅 자원이 요구되는데, 본 연구에서는 분할정복 접근법을 통해 적은 컴퓨팅 자원으로 딥러닝 모델 학습을 위한 충분한 양의 데이터를 확보하는 방법을 소개한다. 제안된 방법은 높은 해상도의 이미지를 분할하고 각 영역에 조건 레이블을 부여한 후, 독립적인 부분 영역과 경계를 학습시켜, 경계 손실이 일관적인 이미지를 생성하도록 유도한다. 이후, 분할된 이미지를 하나로 통합하여, 최종적으로 모델이 고해상도의 이미지를 생성하도록 구성하였다. 실험 결과, 본 연구를 통해 증강된 이미지들은 높은 효율성, 일관성, 품질 및 범용성을 보였다.
With the rapid growth of artificial intelligence, the demand for semiconductors is enormously increasing everywhere. To ensure the manufacturing quality and quantity simultaneously, the importance of automatic defect detection during the packaging process has been re-visited by adapting various deep learning-based methodologies into automatic packaging defect inspection. Deep learning (DL) models require a large amount of data for training, but due to the nature of the semiconductor industry where security is important, sharing and labeling of relevant data is challenging, making it difficult for model training. In this study, we propose a new framework for securing sufficient data for DL models with fewer computing resources through a divide-and-conquer approach. The proposed method divides high-resolution images into pre-defined sub-regions and assigns conditional labels to each region, then trains individual sub-regions and boundaries with boundary loss inducing the globally coherent and seamless images. Afterwards, full-size image is reconstructed by combining divided sub-regions. The experimental results show that the images obtained through this research have high efficiency, consistency, quality, and generality.
1. 서론
2. 관련연구
3. 모델 구조
4. 실험
5. 결론
Acknowledgement
References