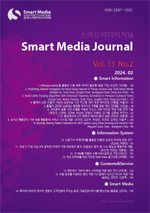
플레이 수준 조절이 가능한 강화학습 기반 카드형 대전 게임 에이전트
Card Battle Game Agent Based on Reinforcement Learning with Play Level Control
- 한국스마트미디어학회
- 스마트미디어저널
- Vol13, No.2
- 2024.02
- 32 - 43 (12 pages)
게임 플레이를 위한 행동 주체인 에이전트는 게임 만족도를 높일 수 있는 중요한 요소이다. 하지만 다양한 게임 난이도와 게임 환경, 여러 플레이어를 위한 게임 에이전트 개발에는 많은 시간과 노력이 필요하다. 또한 캐릭터 추가나 업데이트와 같은 게임 환경 변화가 일어나면 새로운 게임 에이전트의 개발이 필요하고, 개발 난이도는 점차 높아진다는 단점이 존재한다. 이와 함께 다양한 플레이어의 수준에 맞는 세분화된 게임 에이전트 역시 중요하다. 단순히 강한 게임 에이전트보다는 세분화된 수준의 게임 플레이가 가능한 게임 에이전트가 활용성이 높고, 플레이어에 대한 만족도를 높일 수 있기 때문이다. 본 논문에서는 카드형 대전 게임을 대상으로 빠른 게임 에이전트 학습과 세분화된 플레이 수준 조절이 가능한 방법을 제안한다. 제안된 방법은 먼저 행동 구성에 대한 높은 자유도와 멀티 에이전트 환경에서의 빠른 학습을 위해 정책(Policy) 기반 분산형 강화학습 방법 중 하나인 IMPALA를 적용한다. 세분화된 플레이 수준 조절은 Temperature-Softmax를 통해 얻은 행동별 확률 값의 샘플링을 통해 수행한다. 논문에서는 Temperature 값의 증가에 따라 게임 에이전트의 플레이 수준이 낮아지는 결과와 이 수치를 다변화하여 손쉽게 다양한 플레이 수준 조절이 가능함을 확인하였다.
Game agents which are behavioral agent for game playing are a crucial component of game satisfaction. However it takes a lot of time and effort to create game agents for various game levels, environments, and players. In addition, when the game environment changes such as adding contents or updating characters, new game agents need to be developed and the development difficulty gradually increases. And it is important to have a game agent that can be customized for different levels of players. This is because a game agent that can play games of various levels is more useful and can increase the satisfaction of more players than a high-level game agent. In this paper, we propose a method for learning and controlling the level of play of game agents that can be rapidly developed and fine-tuned for various game environments and changes. At this time, reinforcement learning applies a policy-based distributed reinforcement learning method IMPALA for flexible processing and fast learning of various behavioral structures. Once reinforcement learning is complete, we choose actions by sampling based on Softmax-Temperature method. From this result, we show that the game agent's play level decreases as the Temperature value increases. This shows that it is possible to easily control the play level.
Ⅰ. 서론
Ⅱ. 게임 및 학습 환경
Ⅲ. 게임 에이전트 학습
Ⅳ. 플레이 수준 조절
Ⅴ. 실험결과
Ⅵ. 결론