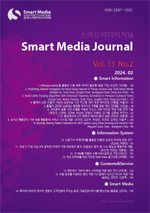
의미론적 분할 기반 모델을 이용한 조선소 사외 적치장 객체 자동 관리 기술
Segmentation Foundation Model-based Automated Yard Management Algorithm
- 한국스마트미디어학회
- 스마트미디어저널
- Vol13, No.2
- 2024.02
- 52 - 61 (10 pages)
조선소에서는 사외 적치장의 관리를 위해 일정 주기로 Unmanned Aerial Vehicle (UAV)을 이용해 항공 영상을 획득하고, 이를 사람이 판독하여 적치장 현황을 파악한다. 이러한 방법은 넓은 면적의 사외 적치장 현황을 파악하는 데 상당한 시간과 인력을 요구한다. 본 논문에서는 이러한 문제점을 해결하고 정확한 사외 적치장 현황을 파악하기 위해 사전 학습된 의미론적 분할 기반 모델(Foundation Model)을 활용한 자동 관리 기술을 제안한다. 또한, 조선소 사외 적치장의 경우 관련 부품이나 장비를 포함한 공개 데이터셋이 충분하지 않기 때문에, 의미론적 분할 기반 모델에 필요한 객체 프롬프트(Prompt)를 생성하기 위한 소규모 사외 적치장 객체 데이터셋을 직접 구축하였다. 이를 이용해 객체 검출기를 소규모 데이터셋에 추가 학습하여 초기 객체 후보를 추출하고, 의미론적 분할 기반 모델인 Segment Anything Model (SAM)의 프롬프트로 활용해 정확한 의미론적 분할 결과를 얻는다. 더 나아가, 지속적인 적치장 데이터셋 수집을 위해 SAM을 활용한 훈련 데이터 생성 파이프라인을 제안한다. 본 연구에서 제안한 방법은 기존의 의미론적 분할 방법과 비교하여 평균적 4.00%p, SegFormer에 비해 5.08%p 높은 성능을 달성하였다.
In the shipyard, aerial images are acquired at regular intervals using Unmanned Aerial Vehicles (UAVs) for the management of external storage yards. These images are then investigated by humans to manage the status of the storage yards. This method requires a significant amount of time and manpower especially for large areas. In this paper, we propose an automated management technology based on a semantic segmentation foundation model to address these challenges and accurately assess the status of external storage yards. In addition, as there is insufficient publicly available dataset for external storage yards, we collected a small-scale dataset for external storage yards objects and equipment. Using this dataset, we fine-tune an object detector and extract initial object candidates. They are utilized as prompts for the Segment Anything Model(SAM) to obtain precise semantic segmentation results. Furthermore, to facilitate continuous storage yards dataset collection, we propose a training data generation pipeline using SAM. Our proposed method has achieved 4.00%p higher performance compared to those of previous semantic segmentation methods on average. Specifically, our method has achieved 5.08% higher performance than that of SegFormer.
Ⅰ. 서론
Ⅱ. 관련 연구
Ⅲ. 제안하는 방법
Ⅳ. 실험 방법 및 결과
Ⅴ. 결론