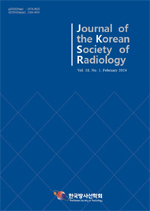
CycleGan 딥러닝기반 인공CT영상 생성성능에 대한 입력 MR영상의 T1 및 T2 가중방식의 영향
Dependency of Generator Performance on T1 and T2 weights of the Input MR Images in developing aCycleGan based CT image generator from MR images
- 한국방사선학회
- 한국방사선학회 논문지
- 제18권 제1호
- 2024.02
- 37 - 44 (8 pages)
MR은 우수한 연조직 대비와 기능 정보를 보여줄 수 있지만, 방사선치료에서 정확한 선량 계산을 위해서는 CT영상의 전자밀도 정보가 필요하다. 방사선치료(Radiotherapy) 계획 워크플로우에서 MR영상과 CT영상을 융합하기 위해 환자는 일반적으로 MR과 CT영상 방식 모두에서 스캔된다. 최근에 딥러닝기술 덕분에 MR영상에서 딥러닝 기반의 CT영상 생성이 가능해졌다. 이로 인해 CT 스캔 작업을 할 필요가 없게 된다. 본 연구에서는 MR영상으로부터 CycleGan 딥러닝 기반 CT영상생성을 구현했다. T1가중이나 T2가중 중에 한가지 또는 그 둘다의 MR영상을 가지고 합습한 3가지의 인공지능 CT생성기를 만들었다. 결과에서 우리는 T1가중 MR 영상 기반으로 학습한 생성기가 T1가중 MR영상이 입력될 때 다른 CT생성기보다 더 나은 결과를 생성할 수 있음을 발견했다. 반면, T2가중 MR영상 기반 CT생성기는 T2가중 MR영상을 입력 받을 때, 다른 시퀀스기반 CT생성기보다 더 나은 결과를 생성할 수 있습니다. MR영상을 기반으로 한 CT생성기는 곧 임상현장에 적용될 수 있는 기술이다. 특정 시퀀스 MR영상으로 학습한 머신러닝 CT생성기는 다른 시퀀스 MR영상으로 학습한 생성기보다 더 그 특정 시퀀스와 같은 MR영상을 입력받을 때 더 나은 CT영상을 생성할 수 있음을 보여주었다.
Even though MR can reveal excellent soft-tissue contrast and functional information, CT is also required for electron density information for accurate dose calculation in Radiotherapy. For the fusion of MRI and CT images in RT treatment planning workflow, patients are normally scanned on both MRI and CT imaging modalities. Recently deep-learning-based generations of CT images from MR images became possible owing to machine learning technology. This eliminated CT scanning work. This study implemented a CycleGan deep-learning-based CT image generation from MR images. Three CT generators whose learning is based on T1- , T2- , or T1-&T2-weighted MR images were created, respectively. We found that the T1-weighted MR image-based generator can generate better than other CT generators when T1-weighted MR images are input. In contrast, a T2-weighted MR image-based generator can generate better than other CT generators do when T2-weighted MR images are input. The results say that the CT generator from MR images is just outside the practical clinics and the specific weight MR image-based machine-learning generator can generate better CT images than other sequence MR image-based generators do.
Ⅰ. INTRODUCTION
Ⅱ. METHODS
Ⅲ. RESULT
Ⅳ. DISCUSSION
Acknowledgement
Reference