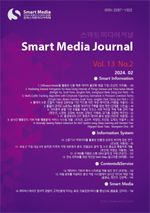
Incorporating BERT-based NLP and Transformer for An Ensemble Model and its Application to Personal Credit Prediction
Incorporating BERT-based NLP and Transformer for An Ensemble Model and its Application to Personal Credit Prediction
- 한국스마트미디어학회
- 스마트미디어저널
- Vol13, No.4
- 2024.04
- 9 - 15 (7 pages)
Tree-based algorithms have been the dominant methods used build a prediction model for tabular data. This also includes personal credit data. However, they are limited to compatibility with categorical and numerical data only, and also do not capture information of the relationship between other features. In this work, we proposed an ensemble model using the Transformer architecture that includes text features and harness the self-attention mechanism to tackle the feature relationships limitation. We describe a text formatter module, that converts the original tabular data into sentence data that is fed into FinBERT along with other text features. Furthermore, we employed FT-Transformer that train with the original tabular data. We evaluate this multi-modal approach with two popular tree-based algorithms known as, Random Forest and Extreme Gradient Boosting, XGBoost and TabTransformer. Our proposed method shows superior Default Recall, F1 score and AUC results across two public data sets. Our results are significant for financial institutions to reduce the risk of financial loss regarding defaulters.
Ⅰ. INTRODUCTION
Ⅱ. PROPOSED METHOD
Ⅲ. EXPERIMENT
Ⅳ. CONCLUSION
REFERENCES