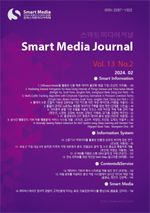
DDPG 및 연합학습 기반 5G 네트워크 자원 할당과 트래픽 예측
5G Network Resource Allocation and Traffic Prediction based on DDPG and Federated Learning
- 한국스마트미디어학회
- 스마트미디어저널
- Vol13, No.4
- 2024.04
- 33 - 47 (15 pages)
향상된 모바일 광대역(eMBB), 초저지연 및 고신뢰 통신(URLLC), 대규모 기계형 통신(mMTC) 등의 특징을 가진 5G의 등장으로 인해 효율적인 네트워크 관리와 서비스 제공을 위해 증가하는 네트워크 트래픽과 복잡성 해결이 시급한 상황이다.본 논문에서는 기계학습(Machine Learning, ML) 및 딥러닝(Deep Learning, DL) 기술을 활용하여 5G 네트워크의 초고속, 초저지연, 초연결성이라는 주요 과제를 해결하면서 네트워크 슬라이싱 및 자원 할당을 동적으로 최적화하는 새로운 접근 방식을 제시한다. 제안된 기법에서는 네트워크 트래픽 및 자원 할당에 대한 예측 모델, 네트워크 대역폭 및 지연 시간을 최적화하면서 동시에 개인 정보와 보안을 향상시키기 위한 연합 학습(FL) 기법을 사용한다. 특히, 본 논문에서는 랜덤 포레스트와 LSTM 등 다양한 알고리듬과 모델의 구현 방법에 대해 자세히 다루며, 이를 통해 5G 네트워크 운영의 자동화와 지능화를 위한 방법론을 제시한다. 마지막으로 제안된 기법을 통해 5G 네트워크에 ML 및 DL을 적용하여 얻을 수 있는 성능향상 효과를 성능평가 및 분석을 통해 검증하고 다양한 산업 응용 분야에서 네트워크 슬라이싱 및 자원 관리 최적화를 위한 솔루션을 제시한다.
With the advent of 5G, characterized by Enhanced Mobile Broadband (eMBB), Ultra-Reliable Low Latency Communications (URLLC), and Massive Machine Type Communications (mMTC), efficient network management and service provision are becoming increasingly critical. This paper proposes a novel approach to address key challenges of 5G networks, namely ultra-high speed, ultra-low latency, and ultra-reliability, while dynamically optimizing network slicing and resource allocation using machine learning (ML) and deep learning (DL) techniques. The proposed methodology utilizes prediction models for network traffic and resource allocation, and employs Federated Learning (FL) techniques to simultaneously optimize network bandwidth, latency, and enhance privacy and security. Specifically, this paper extensively covers the implementation methods of various algorithms and models such as Random Forest and LSTM, thereby presenting methodologies for the automation and intelligence of 5G network operations. Finally, the performance enhancement effects achievable by applying ML and DL to 5G networks are validated through performance evaluation and analysis, and solutions for network slicing and resource management optimization are proposed for various industrial applications.
Ⅰ. 서론
Ⅱ. 관련 연구
Ⅲ. 시스템 모델
Ⅳ. 제안된 모델
Ⅴ. 성능 분석
Ⅵ. 결론
REFERENCES