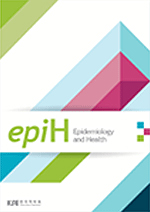
Association of insomnia and daytime napping with metabolic syndrome and its components in a Korean population: an analysis of data from the Korean Genome and Epidemiology Study
Association of insomnia and daytime napping with metabolic syndrome and its components in a Korean population: an analysis of data from the Korean Genome and Epidemiology Study
- 한국역학회
- Epidemiology and Health
- 46
- : SCOPUS, SCIE
- 2024.01
- 1 - 10 (10 pages)
OBJECTIVES: We investigated the association between metabolic syndrome (MetS) and the coexistence of insomnia and daytime napping, because limited data have been reported regarding this association. METHODS: The study population was 8,440 participants aged 40-65 years, who were from the Korean Genome and Epidemiology Study. Self-reported information on insomnia symptoms and nap duration was used to define exposure variables. Data on waist circumference (WC), blood pressure (BP), and fasting blood glucose (FBG), triglyceride (TG), and high-density lipoprotein cholesterol levels in blood were used to define MetS. Multivariate logistic regression analysis was performed to obtain odds ratio (OR) and 95% confidence interval (CI). RESULTS: In multivariate logistic regression analysis, the coexistence of insomnia and napping was not significantly associated with MetS. However, the insomnia and non-napping group showed higher ORs of high TG (OR, 1.19; 95% CI, 1.02 to 1.39) and high BP (OR, 1.28; 95% CI, 1.10 to 1.49) than the non-insomnia and non-napping group. The combination of non-insomnia and napping and that of insomnia and napping showed higher ORs of high TG (OR, 1.13; 95% CI, 1.00 to 1.29) and high FBG (OR, 1.59; 95% CI, 1.14 to 2.21), respectively. In analyses of insomnia symptoms, only the combination of difficulty in maintaining sleep (DMS) and non-napping showed a higher OR for MetS (OR, 1.25; 95% CI, 1.03 to 1.52) than the non-DMS and non-napping group. CONCLUSIONS: Individuals with insomnia, particularly those who do not take naps, were disproportionately likely to have MetS components, especially TG or BP. Information on these variables may help predict individuals’ vulnerability to specific MetS components.
INTRODUCTION
MATERIALS AND METHODS
RESULTS
DISCUSSION
NOTES
REFERENCES