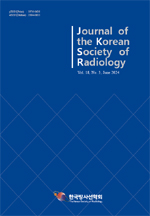
멀티 모달리티 데이터 활용을 통한 골다공증 단계 다중 분류 시스템 개발
Multi-classification of Osteoporosis Grading Stages Using Abdominal Computed Tomography with Clinical Variables : Application of Deep Learning with a Convolutional Neural Network
- 한국방사선학회
- 한국방사선학회 논문지
- 제18권 제3호
- 2024.06
- 187 - 201 (15 pages)
골다공증은 전 세계적으로 주요한 건강 문제임에도 불구하고, 골절 발생 전까지 쉽게 발견되지 않는 단점을 가지고 있습니다. 본 연구에서는 골다공증 조기 발견 능력 향상을 위해, 복부 컴퓨터 단층 촬영(Computed Tomography, CT) 영상을 활용하여 정상-골감소증-골다공증으로 구분되는 골다공증 단계를 체계적으로 분류할 수 있는 딥러닝(Deep learning, DL) 시스템을 개발하였습니다. 총 3,012개의 조영제 향상 복부 CT 영상과 개별 환자의 이중 에너지 X선 흡수 계측법(Dual-Energy X-ray Absorptiometry, DXA)으로 얻은 T-점수를 활용하여 딥러닝 모델 개발을 수행하였습니다. 모든 딥러닝 모델은 비정형 이미지 데이터, 정형 인구 통계 정보 및 비정형 영상 데이터와 정형 데이터를 동시에 활용하는 다중 모달 방법에 각각 모델 구현을 실현하였으며, 모든 환자들은 T-점수를 통해 정상, 골감소증 및 골다공증 그룹으로 분류되었습니다. 가장 높은 정확도를 갖는 모델 우수성은 비정형-정형 결합 데이터 모델이 가장 우수하였으며, 수신자 조작 특성 곡선 아래 면적이 0.94와 정확도가 0.80를 제시하였습니다. 구현된 딥러닝 모델은 그라디언트 가중치 클래스 활성화 매핑(Gradient-weighted Class Activation Mapping, Grad-CAM)을 통해 해석되어 이미지 내에서 임상적으로 관련된 특징을 강조했고, 대퇴 경부가 골다공증을 통해 골절 발생이 높은 위험 부위임을 밝혔습니다. 이 연구는 DL이 임상 데이터에서 골다공증 단계를 정확하게 식별할 수 있음을 보여주며, 조기에 골다공증을 탐지하고 적절한 치료로 골절 위험을 줄일 수 있는 복부 컴퓨터 단층 촬영 영상의 잠재력을 제시할 수 있습니다.
Osteoporosis is a major health issue globally, often remaining undetected until a fracture occurs. To facilitate early detection, deep learning (DL) models were developed to classify osteoporosis using abdominal computed tomography (CT) scans. This study was conducted using retrospectively collected data from 3,012 contrast-enhanced abdominal CT scans. The DL models developed in this study were constructed for using image data, demographic/clinical information, and multi-modality data, respectively. Patients were categorized into the normal, osteopenia, and osteoporosis groups based on their T-scores, obtained from dual-energy X-ray absorptiometry, into normal, osteopenia, and osteoporosis groups. The models showed high accuracy and effectiveness, with the combined data model performing the best, achieving an area under the receiver operating characteristic curve of 0.94 and an accuracy of 0.80. The image-based model also performed well, while the demographic data model had lower accuracy and effectiveness. In addition, the DL model was interpreted by gradient-weighted class activation mapping (Grad-CAM) to highlight clinically relevant features in the images, revealing the femoral neck as a common site for fractures. The study shows that DL can accurately identify osteoporosis stages from clinical data, indicating the potential of abdominal CT scans in early osteoporosis detection and reducing fracture risks with prompt treatment.
Ⅰ. INTRODUCTION
Ⅱ. MATERIAL AND METHODS
Ⅲ. RESULTS
Ⅳ. DISCUSSION
Ⅴ. CONCLUSION
Acknowledgement
Reference