데이터마이닝과 베이지안 기법을 활용한 소규모 지역의 부주의 운전에 의한 교통사고 위험 모형 구축
Building Small Area-level Crash Models Associated with Distracted Driving through Data Mining and Bayesian Approaches: A Case Study in Northern Ohio, U.S.
- 위기관리 이론과 실천
- 한국위기관리논집
- Vol.20 No.3
-
2024.03103 - 116 (14 pages)
-
DOI : 10.14251/crisisonomy.2024.20.3.103
- 23
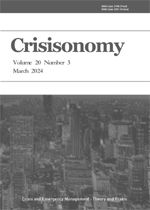
이 연구는 미국 오하이오주 클리블랜드-엘리리아 핵심기반 통계영역의 소규모 지역(센서스 블록그룹)에서 발생한 부주의 운전에 의한 교통사고의 상대위험도에 영향을 미치는 요인 탐색을 위한 연구이다. 이를 위해 공간정보, 사회인구학 및 환경 정보와 교통사고 등을 포함한 다양한 분야의 공공 데이터수집과 데이터 마이닝 기법이 활용되었다. 구축된 자료를 바탕으로 계층적 베이지안 기법을 적용하여일반화선형모형 기반의 교통사고 분석 모형을 개발하였다. 선정된 최적모형에 따르면, 고용과 직장등 사람들의 활동 밀도, 토지이용의 다양성, 교차로 밀도의 경우 부주의 운전에 의한 교통사고의 상대위험을 높이는 것으로 나타났다. 반면, 인구밀도는 교통사고의 위험을 감소시키는 것으로 나타났다. 아울러, 잔차의 불확실성(확률적 변동)의 대부분은 연구 지역 내 블록그룹과 센서스 트랙의 변동성에서 기인한 것으로 나타났다. 이 연구의 결과를 활용하여, 우리는 부주의 운전에 의한 교통사고의 위험 경감을위한 지역의 교통안전 정책 수립에 기여할 수 있을 것이다.
This study aims to identify influential factors affecting the relative risks of vehicle crashes related to distracted driving at small area-levels (i.e., census block group) in Northern Ohio, U.S. To this end, we utilize data mining techniques to analyze publicly available data from multiple sources, which include spatial, sociodemographic, and environmental features alongside crash data. We then develop several generalized linear mixed models using a full Bayesian hierarchical formulation. The selected optimal model indicates that factors such as gross activity density, landuse mix, and density of intersection appear to increase the relative risks of vehicle crashes due to distracted driving, while population density contributes to reducing these risks. In addition, we observe substantial random fluctuation of residuals originating from both block-group and census tract level variability across the study region. These findings allow us to help build regional transportation safety policies to mitigate negative consequences of distracted driving.
Ⅰ. 서론
Ⅱ. 연구방법
Ⅲ. 분석 결과
Ⅳ. 결론
감사의 글
References
(0)
(0)