머신러닝을 통한 관절염 환자의 삶의 질 영향 분석
Analysis of the Impact of Osteoarthritis on Quality of Life Using Machine Learning Method
- 한국자료분석학회
- Journal of The Korean Data Analysis Society (JKDAS)
- Vol.26 No.4
-
2024.081191 - 1203 (13 pages)
-
DOI : 10.37727/jkdas.2024.26.4.1191
- 56
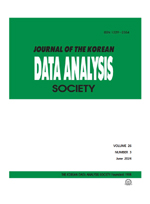
본 연구는 골관절염 환자의 건강 관련 삶의 질을 분석하고 머신러닝을 활용하여 삶의 질을 추정하는 예측 모델을 개발하는 것을 목표로 하였다. 분석 자료는 2019년 국민건강영양조사를 이용하였으며, 관절염이 삶의 질에 미치는 영향을 분석하기 위해 인구 사회학적 요인과 질병 요인 등을 포함한 위계적 회귀분석을 수행하였다. 또한 다중회귀, 라쏘규제회귀(lasso regularized regression), 랜덤포레스트(random forest), XGBoost(extreme gradient boosting)와 같은 머신러닝 기법을 사용하여 예측 모델을 개발하였다. 분석 결과, 총 8,110명의 데이터에서 삶의 질 변수 EQ-5D의 결측값이 있는 19세 미만 대상자를 제외한 5,837명을 최종 분석 대상으로 선정하였고, 이 중 602명이 관절염 유병 군으로 확인되었다. 관절염 유병 군에서 EQ-5D로 측정된 삶의 질은 정상군보다 유의하게 낮았으며, 인구 사회학적 및 질병 이환 변수 등을 포함한 위계적 회귀 분석에서 관절염의 삶의 질에 대한 유의한 영향을 확인하였다. XGBoost(default)에 비교하여 다중회귀와 라쏘회귀 모형, 랜덤포레스트, XGBoost(tuned) 등의 예측 성능은 유의하게 양호한 것으로 나타났으며 네 모델 간의 유의한 차이는 없었다. 랜덤포레스트와 XGBoost의 사후 분석에서 관절염이 주요 변수임을 확인하였으며, 다중회귀 및 라쏘회귀를 사용하여 관절염 환자의 삶의 질 예측 회귀식을 최종 도출하였다. 본 연구는 머신러닝을 활용하여 관절염 환자의 삶의 질을 예측한 최초의 시도이며, 관절염에 대한 새로운 임상 평가 및 중재 효과 평가에 기여할 것으로 사료된다.
This study aimed to analyze the health-related quality of life(HRQoL) of osteoarthritis patients and develop a predictive model using machine learning. Data from the 2019 Korea National Health and Nutrition Examination Survey(KNHANES) were used. Hierarchical regression analysis examined the impact of osteoarthritis on quality of life. Multiple linear regression, lasso regression, random forest and XGBoost were used. Of 8,110 participants, 5,837 were selected, excluding those under 19 with missing EQ-5D data; of these, 602 had osteoarthritis. Their average EQ-5D score was lower than the normal group's. Analysis showed osteoarthritis significantly affects quality of life. Compared to the XGBoost(default), the predictive performance of multiple linear regression, lasso regression, random forest and XGBoost(tuned) models was significantly better, with no significant differences among these. Random forest and XGBoost confirmed osteoarthritis as a key factor. Regression equations to predict quality of life were derived. This study is the first to use machine learning for this purpose and aims to support new clinical assessments and interventions.
1. 서론
2. 연구 방법
3. 연구 결과
4. 논의
5. 결론
References
(0)
(0)