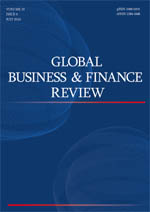
AI-based Pairs Trading Strategies: A Novel Approach to Stock Selection
AI-based Pairs Trading Strategies: A Novel Approach to Stock Selection
- People & Global Business Association
- Global Business and Finance Review
- Vol.29 No.7
- : SCOPUS
- 2024.08
- 1 - 15 (15 pages)
Purpose: This study aims to explore the optimization of Stock Pairs Trading Strategies' performance using AI techniques, with a focus on accurately evaluating stock similarities and selecting the most suitable pairs. Design/methodology/approach: A variety of AI models, including Autoencoders (AE), Vector Embeddings (VE), Recurrent Neural Networks (RNN), Long Short-Term Memory (LSTM), and Gated Recurrent Units (GRU), are utilized to assess the similarity between stocks, which is crucial in the stock pairs selection process for implementing the Pairs Trading strategy. Findings: The implementation of the Pairs Trading strategy with stock pairs selected through AI models showed higher profitability than conventional methods. Strategies utilizing LSTM models demonstrated the highest performance, achieving an approximate cumulative return of 51.25353%. This indicates that AI models are capable of accurately assessing similarities and establishing effective trading strategies. Research limitations/implications: The study highlights the potential of AI-based stock pair selection methods to enhance Pairs Trading Strategies' performance. This approach surpasses traditional statistical methods by better reflecting the stock market's complexity and dynamism, potentially offering investors more stable and higher returns. Originality/value: The research contributes to the field by demonstrating the effectiveness of AI models in the stock pair selection process, suggesting a novel approach to enhancing Pairs Trading Strategies that could provide valuable insights for investors seeking more sophisticated investment strategies in the financial markets.
Ⅰ. Introduction
Ⅱ. Background and Related work
Ⅲ. Methodology
Ⅳ. Research Findings
Ⅴ. Conclusions
References