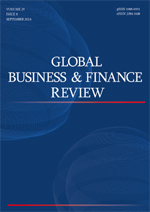
A Comparative Analysis of Deep Learning and Traditional Statistics for Stock Price and Return Forecasting
- People & Global Business Association
- Global Business and Finance Review
- Vol.29 No.8
- : SCOPUS
- 2024.09
- 1 - 17 (17 pages)
Purpose: This study aims to present a comparative analysis of various statistical, machine learning, and deep learn-ing models for predicting stock prices and returns across sectors on the Indonesian Stock Exchange. Design/methodology/approach: The research analyzes two indexes and eight prominent companies from different sectors using historical data of fundamental, technical indicators, economic factors, analyst recommendation, and social media sentiment during period of 2012-2022. Models such as ARIMA, SARIMA, VAR, Random Forests, GBM, SVM, ANNs, RNNs, and LSTM are applied. Findings: The results indicate that the efficacy of forecasting models varies with the sector and the time scale. ANNs and RNNs outperform for daily forecasts, while VAR is more effective for quarterly and yearly forecasts. Traditional statistical models like VAR and SARIMA are effective in the finance sector for daily and yearly fore-casts due to stability and predictability. Machine learning models like GBM and ANN perform better in the mining and infrastructure sectors, which face abrupt changes from external factors. Deep learning models excel in sectors like consumer goods and trade for yearly forecasts, capturing intricate, evolving patterns. Despite the strengths of deep learning models in specific stocks, no single model universally excels across all contexts. Research limitations/implications: The study is limited to the Indonesian Stock Exchange. Further research could expand the scope to other markets. The effectiveness of predictive tools may be reduced in markets with significant information gaps, affecting their accuracy in less efficient markets. Originality/value: This research fills a gap by systematically comparing traditional statistical methods, machine learning, and deep learning models across multiple sectors and timeframes, providing valuable insights into strategic model selection for stock price and return forecasting.
I. Introduction
II. Literature Review
III. Research Methodology
IV. Result
V. Discussion
VI. Conclusion
References