히스토그램 자료를 위한 성긴 k-평균 군집분석에 관한 연구
A study on sparse k-means clustering for histogram-valued data
- 한국자료분석학회
- Journal of The Korean Data Analysis Society (JKDAS)
- Vol.26 No.5
-
2024.101317 - 1329 (13 pages)
-
DOI : 10.37727/jkdas.2024.26.5.1317
- 8
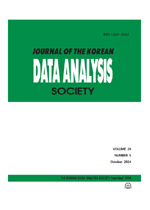
본 논문에서는 대표적인 심볼릭 데이터(symbolic data)인 히스토그램 자료를 위한 성긴 k-평균군집분석에 대해 연구하였다. p차원 히스토그램 자료를 군집화하기 위하여 히스토그램 자료간의 거리를 Wasserstein-Kantorovich 거리를 이용하여 측정하고 p개의 변수에 성긴 k-평균 군집분석(sparse k-means clustering) 알고리즘을 적용하여 변수별 가중치를 구하고 이를 이용하여 군집 결과를 얻는다. 이 방법은 가중치를 적용한 군집간 거리 제곱합을 최대로 하는 가중치를 찾는 군집방법이다. 여러 다른 군집 수에 대하여 성긴 k-평균 군집 알고리즘을 적용하고 실루엣(Silhouette) 측도를 이용하여 이 측도가 최대가 되는 군집 개수를 적정한 군집 개수로 결정한다. 성긴 k-평균 군집분석의 성능을 확인하기 위해 여러 분포에 대해 자료를 생성하여 모의실험을 실시하여 군집의 일치도와 선택되는 변수의 측면에서 k-평균 군집분석과 비교를 하였고 미국의 48개 주 월별 평균 기온자료를 이용해 실제 자료 분석을 실시하였다. 그 결과 제안된 방법은 군집에 필요한 변수를 잘 선택하면서도 군집의 일치도 측면에서 좋은 성능을 보였으며 실제 자료분석에서도 적절한 분석 결과를 보였다.
In this paper, we investigate a sparse k-means clustering method for histogram-valued data. The distances between histogram-valued observations are defined using the Wasserstein-Kantorovich distances to group p-dimensional histogram-valued data. Clustering is performed using the sparse k-means clustering method with the distance matrix computed for each dimension. The proposed method maximizes the weighted sums of squared distances between clusters. For various value of k, we apply the sparse k-means clustering method and determine the optimal number of clusters with the Silhouette measure. Simulation studies were conducted to compare the proposed method with the standard k-means clustering method in terms of cluster agreement and selected variables. Additionally, we analyzed real data from the monthly average temperatures of 48 US states. As a result of numerical analysis, it was confirmed that the proposed method shows superior performance and effective variable selection.
1. 서론
2. 이론적 배경
3. 히스토그램 자료에 대한 성긴 k-평균 군집분석
4. 모의실험 및 자료분석
5. 결론
References
(0)
(0)