학습기에 따른 부스팅 성능 비교
A Comparison of Boosting Performance by Learner
- 한국자료분석학회
- Journal of The Korean Data Analysis Society (JKDAS)
- Vol.26 No.5
-
2024.101331 - 1344 (14 pages)
-
DOI : 10.37727/jkdas.2024.26.5.1331
- 10
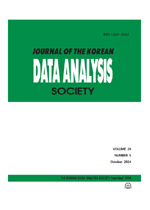
앙상블 학습이란 여러 개의 모형을 결합하여 성능이 강한 모형을 만드는 학습 방법으로 실생활의 많은 분야에서 우수한 성능을 나타내고 있다. 앙상블 학습 방법에는 크게 부스팅과 배깅기법이 있으며, 이 두 방법의 차이는 학습기를 순차적으로 학습시키는지 혹은 독립적으로 학습시키는지에서 발생한다. 대부분의 부스팅 방법론에서는 의사결정나무를 학습기로 하여 우수한 성능을 내고 있으며 이들을 비교하는 논문도 존재한다. 하지만, 의사결정나무가 아닌 다른 모형도 부스팅의 학습기로 활용될 수 있으므로 본 논문에서는 다양한 학습 방법을 적용하여 그 결과를 비교하였다. 이항 분류 문제의 상황으로 한정하여 아다부스트와 그래디언트 부스팅의 두 가지 부스팅 방법론에 일반화 선형 모형, 서포트 벡터 머신, K-최근접 이웃, 판별 분석, 그리고 인공 신경망을 학습기로 적용하여 부스팅의 정확도를 비교하였다. 비교 결과, 전체 여섯 가지의 학습 방법론 중에서 의사결정나무를 기반으로 하는 부스팅의 성능이 가장 우수하였고, 일반화 선형 모형과 K-최근접 이웃, 인공 신경망을 기반으로 하는 부스팅은 대체로 준수한 성능을 보였으며 서포트 벡터 머신과 판별 분석을 기반으로 하는 경우에는 가장 좋지 않은 성능을 보였다.
Ensemble learning is a learning method that combines several models to create a model with strong performance, showing excellent performance in many fields of real life. Ensemble learning methods largely have boosting and bagging techniques, and the difference between these two methods arises from whether the learner is trained sequentially or independently. In most boosting methodologies, decision trees are used as learners to perform well, and there are papers comparing them. However, models other than decision trees can also be used as learners of boosting, thus in this paper, various learning methods were applied and the results were compared. Limited to the situation of binomial classification problems, the accuracy of boosting was compared by applying a generalized linear model, a support vector machine, a K-nearest neighbors, discriminant analysis, and an artificial neural network as learners. As a result, out of all six learning methodologies, boosting based on decision trees was the best, and boosting based on generalized linear models, K-nearest neighbors, and artificial neural networks generally showed good performance, and worst performance based on support vector machines and discriminant analysis.
1. 서론
2. 통계 분석 방법론
3. 실험 설계
4. 실험 결과
5. 결론
References
(0)
(0)