의사결정나무 분석에 대한 국내 연구 동향 탐색
Exploration of domestic research trends on decision tree analysis
- 한국자료분석학회
- Journal of The Korean Data Analysis Society (JKDAS)
- Vol.26 No.5
-
2024.101375 - 1393 (19 pages)
-
DOI : 10.37727/jkdas.2024.26.5.1375
- 221
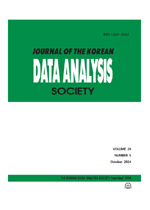
본 연구는 데이터마이닝 기법인 의사결정나무의 국내 연구 동향을 살펴봄으로써 본 분석방법의 기초적인 논리와 알고리즘, 그리고 다양한 활용 가능성을 탐색하는 데 목적이 있다. 이를 위해 2001년~2023년 국내 의사결정나무 분석을 활용한 학술논문 1,075편을 대상으로 연도별, 학술지별, 주제분야별, 저자별 논문 빈도와 논문에서 사용된 알고리즘의 빈도, 키워드 빈도, 키워드 중심성 분석을 실시하였다. 주요 분석 결과, 논문 빈도는 2001년 1편에서 시작하여 최근 2023년 87편으로 점차 증가한 것을 볼 수 있고, 사회과학 분야가 377편(35.07%)으로 가장 많았으며, 자연과학 분야 233편(21.67%), 공학 분야 230편(21.40%) 순으로 나타났다. 사용된 알고리즘은 CHAID가 315회(36.42%)로 가장 많이 활용되었고, CART가 310회(35.84%), 그리고 랜덤포레스트와 같은 앙상블 기법이 124회(14.34%), C5.0이 94회(10.87%)로 나타났으며, Quest의 사용빈도는 10회(1.16%)로 매우 낮았다. 연도별 알고리즘의 사용 흐름을 보면 의사결정나무의 예측률을 높이기 위한 앙상블 기법의 사용이 점차 증가하고 있는 것으로 나타났다. 논문 키워드는 ‘의사결정나무’가 687개, ‘데이터마이닝’ 239개, ‘머신러닝’ 105개, ‘로지스틱’ 98개, ‘신경망’ 68개 순으로 나타났으며, 연구내용과 관련된 키워드로 ‘자살’, ‘우울’, ‘고혈압’, ‘아건강(亚健康)’ 등과 같이 질병이나 건강과 관련된 키워드가 상위 키워드로 도출되어 의사결정나무가 의학 분야와 관련된 연구에서 많이 사용된 것을 알 수 있다. 이와 같은 분석 결과를 토대로 시사점 및 후속 연구를 제안하였다.
This study explores the basic system and applicability of decision tree techniques by examining domestic research trends in this field. We analyzed 1,075 academic papers using domestic decision tree analysis from 2001 to 2023, categorized by year, journal, topic field, author, paper frequency, and frequency of algorithms used. Key findings reveal that the frequency of papers began with one in 2001, increasing to 87 in 2023. The distribution across fields was as follows: social science (377 papers, 35.07%), natural science (233 papers, 21.67%), and engineering (230 papers, 21.40%). Among the algorithms, CHAID was used most frequently (315 times, 36.42%), followed by CART (310 times, 35.84%), ensemble techniques like random forest (124 times, 14.34%), and C5.0 (94 times, 10.87%). The Quest algorithm was rarely used (10 times, 1.16%). The annual usage trends indicate a growing preference for ensemble techniques to enhance decision tree prediction rates. The paper's keywords included “decision trees” (687), “data mining” (239), “machine learning” (105), “logistic” (98), and “neural networks” (68). Notably, keywords related to health, such as “suicide,” “depression,” “high blood pressure,” and “health,” emerged prominently, signifying the extensive use of decision trees in medical research. Based on these findings, we propose implications and directions for follow-up studies.
1. 서론
2. 이론적 배경
3. 연구 방법
4. 연구 결과
5. 결론 및 논의
Reference
(0)
(0)