머신러닝기반 오이 생육 최적 예측 모델에 관한 연구
A Study on the Optimal Forecasting Model for Cucumber Growth Based on Machine Learning
- 한국전자통신학회
- 한국전자통신학회 논문지
- 제19권 제5호
-
2024.10911 - 918 (8 pages)
-
DOI : 10.13067/JKIECS.2024.19.5.911
- 37
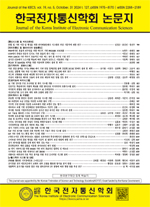
본 연구는 오이 생육 데이터를 활용하여 머신러닝 기반 착과수 예측 모델을 개발하고 성능을 평가하였다. 본 연구에서는 초장, 마디수, 평균절간장, 줄기 굵기, 엽장, 엽폭, 엽수, 암꽃수를 독립 변수로, 착과수를 종속 변수로 설정하여 예측 모델을 개발하였다. 선형 회귀, 랜덤 포레스트, XGBoost, 서포트 벡터 회귀(SVR), K-최근접 이웃(KNN) 등 다양한 머신러닝 알고리즘을 적용하였으며, 모델 성능은 평균 제곱 오차(MSE)와 결정계수(R²)를 기준으로 평가하였다. 그 결과, 랜덤 포레스트 알고리즘이 MSE 3.91, R² 0.828로 가장 우수한 성능을 보였으며, 오이 생육 데이터의 비선형 관계를 효과적으로 포착함을 확인할 수 있었다. 특히, 랜덤 포레스트 모델은 이상치에 대해 강건한 성능을 보였고, 착과수 예측에서 뛰어난 성능을 입증하였다.
This study developed and evaluated the performance of a machine learning-based model for predicting cucumber fruit set using cucumber growth data. In this study, plant height, node number, internode length, stem thickness, leaf length, leaf width, leaf count, and female flower count were used as independent variables, and the fruit set was set as the dependent variable to develop a prediction model. Various machine learning algorithms, including Linear Regression, Random Forest, XGBoost, Support Vector Regression (SVR), and K-Nearest Neighbors (KNN), were applied, and model performance was evaluated based on Mean Squared Error (MSE) and the coefficient of determination (R²). As a result, the Random Forest algorithm demonstrated the best performance, with an MSE of 3.91 and an R² of 0.828, effectively capturing the non-linear relationships in the cucumber growth data. In particular, the Random Forest model showed robustness against outliers and proved to be highly effective in predicting fruit set.
Ⅰ. 서 론
Ⅱ. 관련 연구
Ⅲ. 연구 방법
Ⅳ. 연구 결과
Ⅴ. 결 론
References
(0)
(0)