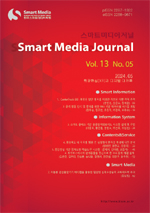
스마트 축사내 상황인지 자율이동형 환경센서 개발 및 가축행동 분석에 관한 연구
A Study on the Development of Autonomous Mobile Environmental Sensors and Livestock Behavior Analysis for Situation Awareness in Smart Barns
- 한국스마트미디어학회
- 스마트미디어저널
- Vol13, No.10
- : KCI등재후보
- 2024.10
- 35 - 42 (8 pages)
본 연구는 스마트 축사 내에서 자율주행 시스템을 활용하여 가축의 행동 패턴과 환경 데이터를 기반으로 소의 질병 유무를 예측하는 시스템을 개발하는 것을 목표로 한다. 외부 기기(RFID 태그 등) 없이 순수 카메라 영상만을 이용해 각 소의 고유 ID를 유지하는 것이 필수적이며, 이를 통해 소별로 시간대에 따른 서기, 앉기, 누워있기 등의 행동 패턴을 추적할 수 있다. 또한, 온도, 습도와 같은 환경 데이터를 통합하여 소의 건강 상태를 종합적으로 평가한다. 이를 위해 YOLO를 이용한 객체 탐지, Deep SORT를 이용한 추적, 그리고 ReID를 이용한 재식별 알고리즘을 결합한 고유 ID 유지 알고리즘을 제안하였다. 실험 결과, YOLO + Deep SORT + ReID 알고리즘이 고유 ID 유지 성능에서 가장 우수한 결과를 보였으며, LSTM 기반 행동 분석 모델이 행동 패턴 예측에서 높은 정확도를 나타내었다. 본 연구의 시스템은 축사 내부의 환경 데이터와 행동 패턴을 종합적으로 분석하여 가축의 질병이나 스트레스 상태를 실시간으로 예측할 수 있는 효과적인 도구로 활용될 수 있을 것이다.
This study aims to develop a system that predicts the health status of cattle based on behavior patterns and environmental data within a smart barn using an autonomous driving system. Maintaining a unique ID for each cow using only a camera, without external devices (such as RFID tags), is essential. This enables the tracking of behavior patterns such as standing, sitting, and lying for each cow over time. Additionally, environmental data such as temperature and humidity are integrated to comprehensively assess the cows' health conditions. To achieve this, we propose a unique ID retention algorithm that combines object detection using YOLO, tracking with Deep SORT, and re-identification (ReID). Experimental results show that the YOLO + Deep SORT + ReID algorithm delivers the best performance in maintaining unique IDs, and the LSTM-based behavior analysis model demonstrates high accuracy in predicting behavior patterns. This system can serve as an effective tool for real-time prediction of livestock health conditions, such as disease or stress, through comprehensive analysis of environmental data and behavior patterns inside the barn.
Ⅰ. 서론
Ⅱ. 기존 연구 및 이론적 배경
Ⅲ. 실험설계 및 방법론
Ⅳ. 실험 결과 및 분석
Ⅴ. 결론
REFERENCES