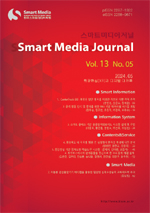
Adversarial Sample Generation and Training using Neural Network
- 한국스마트미디어학회
- 스마트미디어저널
- Vol13, No.10
- : KCI등재후보
- 2024.10
- 43 - 49 (7 pages)
The neural network classifier is known to be susceptible to adversarial attacks, where projected gradient descent-like noise is added to the data, causing misclassification. These attacks can be prevented by min-max training, where the neural network is trained to handle adversarial attack data. Although min-max training is very effective, it requires a large amount of training time because each adversarial attack data generation requires several iterations of gradient back-propagation to produce. In this paper, convolutional layers are used to replace the projected gradient descent-based production of adversarial attack data in an attempt to reduce the training time. By replacing the adversarial noise generation with the output of convolutional layers, the training time becomes comparable to that of a simple neural network classifier with a few additional layers. The proposed approach significantly reduced the effects of smaller-scale adversarial attacks, and under certain circumstances, was shown to be as effective as min-max training. However, for severe attacks, the proposed approach was not able to compete with modern min-max-based remedies.
Ⅰ. INTRODUCTION
Ⅱ. Background
Ⅲ. Proposed Method
Ⅳ. Evaluation
Ⅴ. Conclusion
REFERENCES