A Study on Comparative Analysis of Object Detection-based Real-Time Wildfire and Class B Fire Detection Performance
- 한국화재소방학회
- International Journal of Fire Science and Engineering
- Vol. 38, No. 2
-
2024.061 - 11 (11 pages)
-
DOI : 10.7731/KIFSE.12dac838
- 17
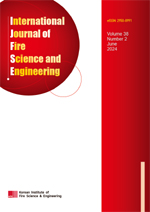
Wildfires cause human casualties, property damage, and significant damage to ecosystems, so prompt fire detection and response are essential. In this study, training and evaluation experiments were performed on the YOLOv3, Faster R-CNN, and Cascade R-CNN models to analyze their real-time wildfire detection performance. The performance of each model was evaluated using precision, recall, mAP 50, mAP 50-95, the model parameter, and frames per second (FPS). The experiment results showed that YOLOv3 had slightly lower overall performance than other models, but the difference was not significant in terms of mAP 50. YOLOv3 had the highest inference speed (48.7 FPS) and an overall mAP 50 of 0.832. On the contrary, Faster R-CNN's overall mAP 50 was 0.835, while Cascade R-CNN showed higher performance with 0.840. Compared to YOLOv3, both models showed relatively low inference speeds (36.2 and 26.3 FPS, respectively). Therefore, the YOLOv3 model is deemed appropriate for real-time fire detection owing to its high inference speed and insignificant wildfire detection performance difference in terms of mAP 50. Future wildfire detection models are expected to improve by optimizing the network structures (e.g., FPN and Head) of 1-Stage models, such as YOLOv3.
1. Introduction
2. Real-Time Object Detection Model
3. Experiment
4. Experiment Results
5. Conclusions
Author Contributions
Conflicts of Interest
Acknowledgments
References
(0)
(0)