Leveraging distributed and parallel algorithm for normalized PCA in hyperspectral image analysis
- 한국자료분석학회
- Journal of The Korean Data Analysis Society (JKDAS)
- Vol.26 No.6
-
2024.121649 - 1659 (11 pages)
-
DOI : 10.37727/jkdas.2024.26.6.1649
- 4
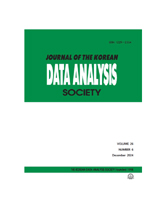
In recent years, the availability of hyperspectral data has rapidly expanded across diverse fields of study. These data, measured by hundreds of spectral bands, are characterized by high dimensionality, posing significant challenges for analysis. Principal Component Analysis (PCA) is a widely-used method for dimensionality reduction. However, sample principal components (PCs) are generally sensitive to difference in scale, and thus hyperspectral datasets with varying scales require proper normalization. Furthermore, the massive volume of hyperspectral data necessitates adopting distributed and parallel computing platforms. In this article, we propose a single-phase MapReduce-based algorithm for normalized PCA on large-scale high-dimensional hyperspectral data by leveraging the distributed and parallel architecture of RHadoop. Our experiment on the Indian Pines dataset underscores the substantial impact of the normalization process on PCA-based feature selection and subsequent machine learning analyses. Applying our proposed algorithm to the large-scale HySpecNet-11k benchmark dataset validates its scalability and efficiency.
1. Introduction
2. Normalization Techniques for Hyperspectral PCA
3. Single-Phase MapReduce Algorithm for Normalized PCA
4. Normalization Effects on PCA-based Machine Learning Performance
5. Scalability Testing with HySpecNet-11k: a Large-Scale Case Study
6. Conclusion
References
(0)
(0)