워드 임베딩 방법에 따른 가짜뉴스 판별 모델에 관한 연구
A study on a fake news identification model based on word embedding method
- 한국자료분석학회
- Journal of The Korean Data Analysis Society (JKDAS)
- Vol.26 No.6
-
2024.121847 - 1853 (7 pages)
-
DOI : 10.37727/jkdas.2024.26.6.1847
- 127
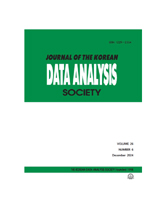
정보화 기술과 미디어화의 가속화 속에 가짜뉴스가 우리 사회의 심각한 문제로 대두되고 있다. 이러한 사회현상에 대응하여 뉴스가 가짜뉴스인지 아닌지를 판별할 수 있는 모델을 개발하여 가짜뉴스 노출에 취약한 현시점에서 사회적으로 유해한 영향을 줄이고 신뢰성 있는 정보 전달에 기여하고자 한다. 자연어를 처리하는 방법 중 단어 임베딩 방법을 기반으로 한 딥러닝 모델을 통해 가짜뉴스를 판별해 내고 그 모델의 성능을 높이고자 다양한 워드 임베딩 방법을 소개하고자 한다. 워드 임베딩 방법은 뉴스 텍스트 데이터에서 의미 있는 특징을 추출하고, 단어 간 의미적 유사성을 파악하는 방법인데, 이 방법을 활용하여 뉴스 기사의 실제 내용과 일치하지 않는 정보를 식별하여 가짜뉴스를 판별하고자 한다. 각 워드 임베딩 방법인 TF-IDF, Word2Vec, FastTextt의 임베딩 행렬을 생성하고, 가짜뉴스 판별 모델인 딥러닝 기반 LSTM 모델에 임베딩층의 가중치를 적용한 후, 모델의 정확도(accuracy)를 비교하여 더 우수한 워드 임베딩 방법을 제시하였다. 본 연구의 사례 분석을 통해 모델의 정확도를 비교한 결과 Word2Vec 방법이 TF-IDF와 FastText보다 더 우수한 결과를 얻었다.
Status of information technology and mediaization Fake news is emerging as a permanent problem in our society. By developing a model to detect fake news, we aim to deliver reliable information about the impact of the current coverage of fake news. Among natural language processing methods, we would like to introduce various embedding methods to share fake news and share the performance of the model through a deep learning model based on the word embedding method. The push embedding method is a method of extracting meaningful features from news text data and identifying meaning and consistency between words. This method is used to identify information that does not match the actual content of the news article and place importance on fake news. After generating the embedding matrix of each word embedding method, TF-IDF, Word2Vec, and FastTextt, and combining the embedding layer with the deep learning-based LSTM model, which is a model with fake news, the power (accuracy) of the model is compared to see which is superior. An embedding method was presented. Comparing the cooperation of the models across participants in this study, we show that the Word2Vec method outperforms TF-IDF and FastText.
1. 서론
2. 자연어 처리 방법
3. 사례 분석
4. 결론 및 토의
References
(0)
(0)