LightGBM 기반 교량 상부구조 부재 성능 예측 모델
Bridge Superstructure Component Performance Prediction Model Based on LightGBM
- 한국방재학회
- 2. 한국방재학회 논문집
- 24권 6호
-
2024.12253 - 261 (9 pages)
-
DOI : 10.9798/KOSHAM.2024.24.6.253
- 21
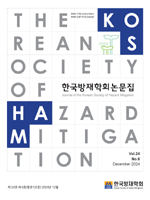
국내 노후화 교량의 급격한 증가로 인해 효율적인 유지관리 전략을 수립할 필요가 있으며, 이때 교량의 성능 예측 모델이 활용될 수 있다. 본 연구에서는 BMS (Bridge Management System)에서 관리하는 데이터를 활용하여 상부구조 중 데이터 수가 많은 부재들(콘크리트 바닥판, PSC I거더, 강박스 거더, 콘크리트 가로보, 강재 가로보)의 성능 예측 모델을 개발하였다. 이전 점검에서의 상태 등급(이전 등급)이 예측에 가장 큰 영향을 미치는 것을 확인하였으며, 회귀분석을 통해 이전 등급을 대푯값이 아닌 회귀값으로 학습시켰다. 그 결과, 학습 모델의 예측 성능이 향상되었으며, 다른 상부구조 부재에 대해서도 이와 같은 방법으로 학습을 진행하였다. 최종적으로 실제 교량 데이터를 적용하여 예측 결과를 분석하였다.
The rapid increase in the number of aging bridges domestically requires efficient maintenance strategies, which may utilize bridge performance prediction models. In this study, performance prediction models were developed for structural components with large amounts of data in the superstructure (concrete decks, PSC I girders, steel box girders, concrete crossbeams, and steel crossbeams) using data managed by a Bridge Management System. The condition rating from previous inspections (previous rating) had the greatest impact on the predictions, and regression analysis was used to train the model with the regression value of the previous rating rather than its representative value. Consequently, the prediction performance of the learning model improved, and the same method was applied to other superstructure components. Finally, the prediction results were analyzed by applying actual bridge data to the model.
1. 서 론
2. 데이터 및 방법론
3. 결과 및 테스트
4. 결 론
감사의 글
References
(0)
(0)