KoBERT 기반 비속어 검출 모델 및 FAST API 서버 구현
Implementation of KoBERT-based profanity detection model and FAST API server
- 한국전자통신학회
- 한국전자통신학회 논문지
- 제19권 제6호
-
2024.121147 - 1152 (6 pages)
-
DOI : 10.13067/JKIECS.2024.19.6.1147
- 75
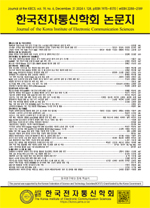
본 논문에서는 한국어 BERT(KoBERT)를 전이 학습하여 비속어가 포함된 문장과 그렇지 않은 문장을 구별하는 모델을 구축하고, 이를 Python의 FAST API를 이용하여 웹 서비스 형태로 구현한 연구 결과를 제시한다. 데이터 셋은 다양한 온라인 커뮤니티와 소셜 미디어에서 수집한 문장을 활용하였으며, 전처리 과정을 거쳐 비속어 여부로 라벨링 하였다. KoBERT를 기반으로 한 분류 모델을 구축하고, 전이 학습 기법을 통해 높은 정확도의 비속어 검출 성능을 달성하였다. 또한, FAST API를 이용하여 클라이언트로부터 POST 요청을 받아 텍스트 데이터를 처리하고, 비속어 여부를 반환하는 웹 서비스를 구현하였다. 본 연구는 KoBERT를 활용한 비속어 검출의 가능성을 확인하고, 실용적인 웹 서비스 구현을 통해 실제 적용 가능성을 제시하였다. 향후 연구로는 더 다양한 데이터 셋을 활용한 모델 성능 개선과 실시간 비속어 필터링 시스템 구현을 목표로 한다.
This paper presents a study in which a model is built to distinguish between sentences containing profanity and those that do not, by applying transfer learning to KoBERT (Korean BERT). The model is implemented as a web service using Python’s FAST API. The dataset consists of sentences collected from various online communities and social media platforms, and after a preprocessing stage, the sentences were labeled based on the presence of profanity. A classification model was built using KoBERT, and by utilizing transfer learning techniques, high accuracy in profanity detection was achieved. Additionally, a web service was implemented using FAST API, which processes text data received through POST requests from clients and returns whether profanity is present or not. This study confirms the potential of using KoBERT for profanity detection and demonstrates the feasibility of practical application through the implementation of a web service. Future research will aim to improve model performance by utilizing more diverse datasets and to implement a real-time profanity filtering system.
Ⅰ. 서 론
Ⅱ. 선행연구
Ⅲ. 설 계
Ⅳ. 구 현
Ⅴ. 검 증
Ⅵ. 결 론
References
(0)
(0)