딥 러닝을 통해 스마트 그리드 이상 탐지 기능 강화
Enhancing Smart Grid Anomaly Detection Through Deep Learning
- 한국전자통신학회
- 한국전자통신학회 논문지
- 제19권 제6호
-
2024.121215 - 1224 (10 pages)
-
DOI : 10.13067/JKIECS.2024.19.6.1215
- 16
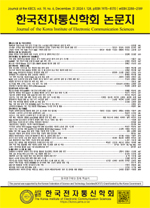
스마트 그리드로의 전력망 현대화는 효율성, 신뢰성, 지속 가능성 측면에서 많은 이점을 제공합니다. 그러나 복잡하고 상호 연결된 스마트 그리드 시스템은 이상 탐지와 그리드 회복력 유지에 있어 새로운 과제를 제시한다. 기존의 이상 탐지 방법은 스마트 그리드 데이터의 동적이고 이질적인 특성에 적응하기 어려워, 이상 탐지 및 완화에 있어 비효율성을 초래한다. 이러한 문제를 해결하기 위해 본 연구는 TensorFlow 프레임워크를 활용한 딥러닝 기술을 통해 스마트 그리드 이상 탐지와 회복력을 향상하는 새로운 접근 방안을 제안한다. 연구의 목표는 두 가지로 나뉜다. 첫째, 스마트 그리드 데이터 내에서 이상을 정확하게 감지할 수 있는 고급 딥러닝 모델을 개발하고, 둘째, 감지된 이상에 대해 선제적으로 대응하고 이를 완화하여 그리드 회복성을 강화하는 것이다.
Modernizing the power grid to a smart grid offers many benefits in terms of efficiency, reliability, and sustainability. However, complex and interconnected smart grid systems present new challenges in detecting anomalies and maintaining grid resilience. Existing anomaly detection methods have difficulty adapting to the dynamic and heterogeneous characteristics of smart grid data, resulting in inefficiency in anomaly detection and mitigation. To solve these problems, this study proposes a new approach to improve smart grid anomaly detection and resilience through deep learning technology using the TensorFlow framework. The goals of the research are divided into two. First, to develop an advanced deep learning model that can accurately detect anomalies within smart grid data, and second, to strengthen grid resilience by proactively responding to and mitigating detected anomalies.
Ⅰ. Introduction
Ⅱ. Methodology
Ⅲ. Experiments and results
Ⅳ. Future work
V. Conclusion
References
(0)
(0)