다중 헤드 어텐션과 결합한 convLSTM을 활용한 군중 밀도 예측
Crowd Density Prediction Using convLSTM Combined with Multi-Head Attention
- 한국전자통신학회
- 한국전자통신학회 논문지
- 제19권 제6호
-
2024.121319 - 1326 (8 pages)
-
DOI : 10.13067/JKIECS.2024.19.6.1319
- 9
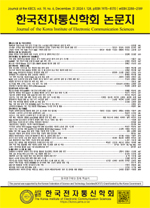
정확한 군중 밀도 예측은 군중 관리, 공공 안전, 도시 계획과 같은 응용 분야에 매우 중요한다. 기존 방법은 비디오 시퀀스의 시간적 종속성과 동적 변화를 포착하는 데 어려움을 겪는 경우가 많아 예측의 신뢰성이 떨어진다. 밀집되고 역동적인 군중은 폐색, 모션 블러 및 다양한 관점을 포함하여 정확한 밀도 추정을 복잡하게 만드는 추가적인 문제를 제시한다. 이러한 문제를 해결하기 위해 우리는 convLSTM(convolutional Long Short-Term Memory) 네트워크를 multi-head attention 메커니즘과 통합하는 새로운 접근 방식을 제안한다. 이 결합된 모델은 복잡한 시나리오에서도 시간적 패턴과 공간적 관계를 효과적으로 캡처하여 예측 정확도를 향상시킨다. 광범위한 실험을 통해 우리의 제안방법이 기존 기술보다 훨씬 뛰어나며 실제 응용 분야에서 정확한 군중 밀도 예측을 위한 강력한 솔루션을 제공한다는 것을 보여준다.
Accurate crowd density prediction is crucial for applications such as crowd management, public safety, and urban planning. Traditional methods often struggle with capturing temporal dependencies and dynamic changes in video sequences, leading to less reliable forecasts. Dense and dynamic crowds present additional challenges, including occlusions, motion blur, and varied perspectives, which complicate accurate density estimation. To address these issues, we propose a novel approach that integrates convolutional Long Short-Term Memory (convLSTM) networks with Multi-Head Attention mechanisms. This combined model improves prediction accuracy by effectively capturing temporal patterns and spatial relationships, even in complex scenarios. Extensive experiments show that our method significantly outperforms traditional techniques, offering a robust solution for precise crowd density prediction in real-world applications.
Ⅰ. INTRODUCTION
Ⅱ. RELATED WORKS
Ⅲ. PROPOSED CROWD DENSITY PREDICTION FRAMEWORK
Ⅳ. Experimental Results
Ⅴ. Conclusion
References
(0)
(0)