Mask R-CNN 알고리즘의 의미론적 분할에 대한 마스크 기반의 성능 평가
Mask-based Performance Evaluation of the Mask R-CNN Algorithm for Semantic Segmentation
- 한국전자통신학회
- 한국전자통신학회 논문지
- 제19권 제6호
-
2024.121327 - 1334 (8 pages)
-
DOI : 10.13067/JKIECS.2024.19.6.1327
- 7
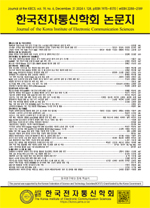
일반적으로 빛, 장애물, 그림자, 곡선 차선 등의 환경적 영향으로 인해 차선 표시를 감지하는 데 어려움이 많고 이로 인해 차선 표시를 감지하는 오류가 높아진다. 본 논문은 환경 조건에 따른 차선 인식의 문제를 해결하기 위해 실제 운전 차량에서 수집한 데이터로부터 Mask R-CNN 알고리즘을 사용하여 의미론적 분할을 수행하고 주행 가능한 영역을 추출하는 방법을 제안한다. 그리고 학습 모델의 성능은 마스크 기반의 평가로 이미지에서 특정 객체의 정확한 위치와 픽셀 수준에서 객체의 모양과 경계에 대한 추정을 평가한다. 실험 결과 낮에 많은 비가 오고 안개가 발생한 경우 주행 가능한 영역 인식률이 높았고, 맑은 날씨이지만 조명이 아주 어두워 앞을 인식하기 어려운 조건에도 인식률이 높았다. 그러나 밤에 폭우가 내리는 경우는 차선 자체인식이 어렵고, 반대 차선의 빛으로 인한 왜곡으로 주행 가능한 영역 인식에 오류 발생률이 높았다.
In general, it is difficult to detect lane markings due to environmental influences such as light, obstacles, shadows, and curved lanes, which increases the error in detecting lane markings. In this paper, we propose a method to perform semantic segmentation and extract drivable areas using the Mask R-CNN algorithm from data collected from actual driving vehicles to solve the problem of lane recognition according to environmental conditions. And the performance of the learning model is evaluated based on the mask-based evaluation to estimate the exact location of a specific object in the image and the shape and boundary of the object at the pixel level. The experimental results showed that the recognition rate of the drivable area was high when it rained heavily during the day and was foggy, and the recognition rate was high even when it was difficult to recognize the front on a clear night in condition of very dark. However, in case of heavy rain night, it was difficult to recognize the lane itself, and the error rate in recognizing the drivable area was high due to distortion caused by the light from the opposite lane.
Ⅰ. 서 론
Ⅱ. 관련 연구
Ⅲ. 방법 및 모델 학습
Ⅳ. 구현 결과 및 분석
Ⅴ. 결 론
References
(0)
(0)