드론 영상 활용 딥러닝 기반 피플 카운팅 알고리즘 개발
Development of a Deep-Learning Based People Counting Algorithm Using Drone Capturing Images
- 한국산업기술융합학회(구. 산업기술교육훈련학회)
- 산업기술연구논문지
- 제29권 4호
-
2024.12175 - 186 (12 pages)
-
DOI : 10.29279/jitr.k.2024.29.4.175
- 25
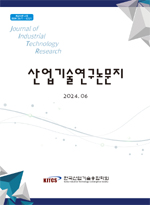
본 연구는 드론 영상을 기반으로 한 학습 데이터를 구축하고 딥러닝을 적용하여 객체를 검출하고 그 수를 카운트하는 알고리즘을 개발하여 드론의 한 분야가 아닌 다양한 분야에 활용될 수 있도록 하는 것을 목표로 한다. 실시간객체 알고리즘인 YOLO를 사용하여 학습을 진행하였고, 이를 바탕으로 객체 검출 성능을 검증하였다. 라벨링 영역을 정교하게 수정하고 그림자가 포함된 학습 데이터를 추가 수집하여 학습을 진행시켜 식별 오류를 해결하였고 90% 이상의 검출 정확도를 확보하였다. 또한, 검출된 객체를 카운트하는 알고리즘을 개발하여 영상 내에 적용한 결과 100% 카운트 성능을 확보하였다. 본 논문에서 제안한 알고리즘을 통해 다중밀집사고 예방뿐만 아니라 사람의 동선파악과 출입 관리 등 다양한 분야에 적용할 수 있고 더 나아가 다양한 환경과 유형의 학습 데이터를 확보한다면실종자 수색 등 재난관리 분야의 넓은 범위에 활용할 수 있을 것으로 판단된다.
This study proposes an algorithm that can be utilized in various drone-related fields. The algorithm builds training data based on drone images and applies deep learning to detect and count the objects. The real-time detection algorithm YOLO was used for training, and the results obtained were used to validate object detection performance. To address identification errors, the labeling area was refined, and additional training data containing shadows were collected, whereby an accuracy of more than 90% was achieved. Furthermore, by developing a people counting algorithm, the proposed method can be applied to various fields, such as crowd crush prevention, tracking people’s movements, and access management. In addition, if diverse environments and types of training data are secured, this algorithm can be widely applied to disaster management fields such as searching for missing persons
Ⅰ. 서 론
Ⅱ. 이론적 배경
Ⅲ. 시스템 설계
Ⅳ. 성능 평가
Ⅴ. 결 론
ACKNOWLEDGEMENTS
References
(0)
(0)