영상 기반 드론 및 UAM 비상 착륙 알고리즘 개발
Development of Emergency Landing Algorithm for Drone and UAM Based on Visual Image Processing
- 한국산업기술융합학회(구. 산업기술교육훈련학회)
- 산업기술연구논문지
- 제29권 4호
-
2024.12187 - 201 (15 pages)
-
DOI : 10.29279/jitr.k.2024.29.4.187
- 39
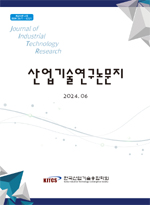
본 연구는 드론 영상을 기반으로 학습 데이터를 구축하고, 딥러닝 기술을 활용하여 객체 추론을 수행하여 착륙가능지점을 추론하고, 해당 지점으로 비상 착륙장소로 선정하는 알고리즘을 개발하였다. 이 알고리즘은 드론과 UAM(Urban Air Mobility) 등 다양한 기체에 적용할 수 있도록 설계되었다. 실시간 객체 탐지 알고리즘인 YOLO-Segmentation 모델을 사용하여 학습을 진행하였으며, Segmentation을 통해 착륙 가능지점에 대한 정확한 경계선 분할을 하여, 이를 기반으로 정확한 영역 탐지와 착륙 가능지점에 대한 검출 성능을 검증하였다. 또한, 여러 시간대에 촬영된 학습 데이터를 통해 다양한 환경적 요인을 고려하여 성능을 향상하였으며, 착륙 가능지점에 대한세그먼트 검출 정확도는 신뢰도 기준 84~98%에 도달하는 성과를 보였다. 본 논문에서 제안한 알고리즘은 다양하고넓은 범위에 활용할 수 있을 것으로 기대된다.
In this study, we developed an algorithm that utilizes drone footage to construct training data, applies deep learning techniques for object inference, identifies suitable landing zones, and designates these zones as emergency landing sites. In particular, we developed a drone-based algorithm using YOLO-Seg deep learning to identify emergency landing zones for drones and UAM vehicles. By incorporating diverse training data, the algorithm segments landing areas with an accuracy of 84%-98% under various conditions. For the training process, we employed the YOLO-Segmentation model, which is a real-time object detection algorithm, to achieve precise boundary segmentation for landing zones. This segmentation facilitates accurate area detection and validates the performance of the algorithm. Furthermore, training data captured at different times of the day were incorporated to account for various environmental factors, thereby enhancing the robustness of the algorithm. This versatile algorithm can be applied to a wide range of aerial mobility safety scenarios. The proposed algorithm has demonstrated application potential in diverse and extensive scenarios related to aerial mobility safety.
Ⅰ. 서 론
Ⅱ. 이론적 배경
Ⅲ. 시스템 설계
Ⅳ. 실험 결과
Ⅴ. 결 론
ACKNOWLEDGEMENTS
References
(0)
(0)