Predicting Working Memory Capacity in Older Subjects Using Quantitative Electroencephalography
- 대한신경정신의학회
- Psychiatry Investigation
- 제15권 제8호
-
2018.08790 - 795 (6 pages)
- 0
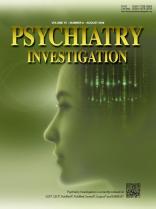
Objective_We utilized a spectral and network analysis technique with an integrated support vector classification algorithm for the automated detection of cognitive capacity using resting state electroencephalogram (EEG) signals. Methods_An eyes-closed resting EEG was recorded in 158 older subjects, and spectral EEG parameters in seven frequency bands, as well as functional brain network parameters were, calculated. In the feature extraction stage, the statistical power of the spectral and network parameters was calculated for the low-, moderate-, and high-performance groups. Afterward, the highly-powered features were selected as input into a support vector machine classifier with two discrete outputs: low- or high-performance groups. The classifier was then trained using a training set and the performance of the classification process was evaluated using a test set. Results_The performance of the Support Vector Machine was evaluated using a 5-fold cross-validation and area under the curve values of 70.15% and 74.06% were achieved for the letter numbering task and the spatial span task. Conclusion_In this study, reliable results for classification accuracy and specificity were achieved. These findings provide an example of a novel method for parameter analysis, feature extraction, training, and testing the cognitive function of elderly subjects based on a quantitative EEG signal.
INTRODUCTION
METHODS
RESULTS
DISCUSSION
(0)
(0)