운고계 자료를 이용하여 기상 현상을 탐지하기 위한 기계학습 기법 적용
Application of Machine Learning to Detect Meteorological Phenomena Using Ceilometer Data
- 인문사회과학기술융합학회
- 예술인문사회융합멀티미디어논문지
- 8권 7호
-
2018.07739 - 748 (10 pages)
- 32
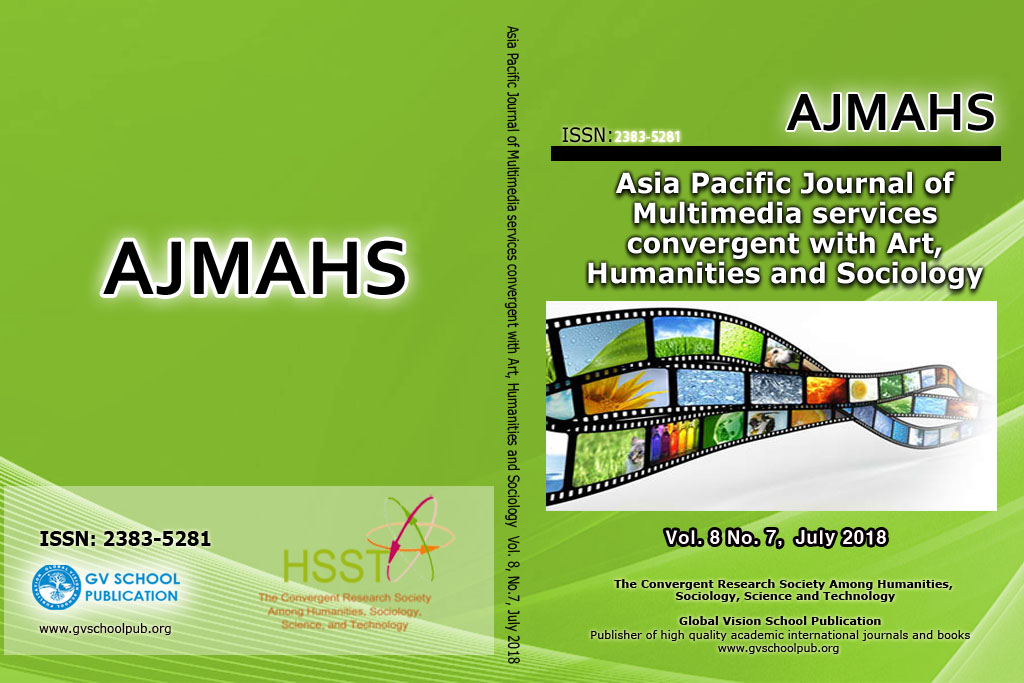
구름은 기후와 날씨 변화에서 가장 중요한 요인 중 하나로, 운고계는 구름의 고도와 운량을 자동으로 관측하는데 사용된다. 본 논문에서는 운고계에서 수집한 후방산란자료에 기계학습기법을 적용하여 기상현상을 탐지하는 방법을 제안한다. 먼저, 수집한 후방산란자료에 잡음을 제거하기 위한 방법으로 선형보간법과 잠음제거 오토인코더를 이용하여 잡음소거를 수행한다. 또한, 관측되는 기상현상이 현저히 적기 때문에 언더샘플링을 적용하여 기계학습기법을 적용했다. 적용한 기법으로는 랜덤포레스트, 서포트 벡터 머신, 인공신경망이며, 학습 시간의 문제로 서포트 벡터 머신과 인공신경망의 경우 특징선택으로 학습인자를 줄여 실험을 진행했다. 탐지한 기상현상으로는 강수, 안개, 기압을 다루었으며 지역별상세관측자료(AWS)와 시정자료를 사용했다. 기계학습의 성능평가를 위한 정확도 측도로 F1-점수를 사용했으며 실험에서 강수의 경우 0.3377, 안개의 경우 0.0949, 기압의 경우 0.3494를 보인다.
Clouds are one of the most important factors in climate and weather changes, and A ceilometer is used to automatically observe information about cloud altitude and cloudiness. In this paper, we propose a method to detect the presence-or-not of meteorological phenomena by applying machine learning to the back-scattered data collected from the ceilometer. First, to eliminate the noise in the observation data, linear interpolation and denoising autoencoder is used to perform noise elimination on the back-scattered data. Since the meteorological phenomena is remarkably small, the machine learning method was applied after undersampling. The machine learning used are Random Forests, Support Vector Machine, and Artificial Neural Network. In case of support vector machine and artificial neural network due to learning time problems, experiments were performed by reducing learning factors by feature selection. We deal with precipitation, fog, and atmospheric pressure as meteorological phenomena using AWS and visibility data. The F1-score was used as an accuracy measure for the performance evaluation of machine learning. In the experiment, it is 0.3377 for the precipitation, 0.0949 for the fog, and 0.3494 for the atmospheric pressure.
1. 서론
2. 기계학습 기법
3. 기상 관측자료
4. 운고계 자료에서 기상현상 탐지
5. 결론
(0)
(0)