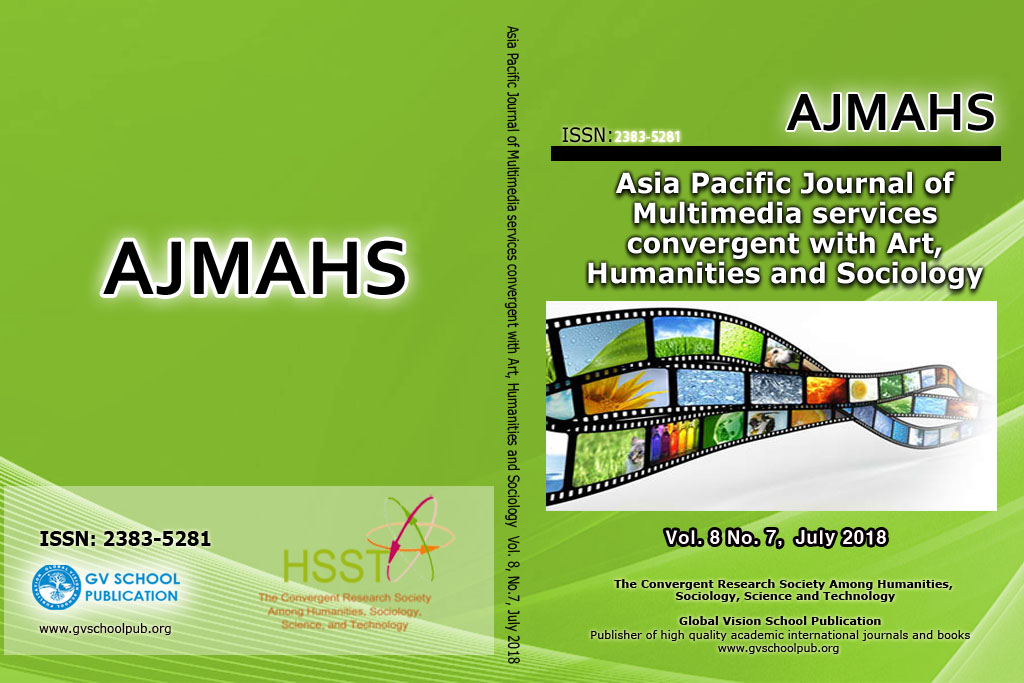
심층 신경망을 이용한 윈드시어 경보 사전 예측
Forecast of Wind-Shear Alert Using Deep Neural Networks
- 인문사회과학기술융합학회
- 예술인문사회융합멀티미디어논문지
- 8권 7호
- 2018.07
- 749 - 757 (9 pages)
항공기의 이륙·착륙에 가장 많은 영향을 끼치는 것 중에 하나는 윈드시어이다. 윈드시어는 wind(바람)과 shear(자르다)가 결합된 용어로, 짧은 수평·수직 거리 내에서 바람의 방향과 속도가 갑자기 변하는 현상을 말한다. 이착륙 단계의 낮은 고도에서는 조종사가 대응할 만한 시·공간적 여유가 없기 때문에, 윈드시어가 발생하면 매우 위험한 상황이 발생할 수 있다. 본 연구에서는 딥 러닝을 이용하여 일정 시간(10분, 20분, 30분) 이후의 윈드시어 발생 경보를 사전에 예측하는 모델을 제안한다. 딥러닝 기법 중의 심층 신경망을 이용하여 모델을 설계하고, 오픈소스 라이브러리인 텐서플로로 구현하였다. 항공기상 관측 장비로 수집된 풍향, 풍속, 온도와 습도 등의 인자를 포함한 인천공항의 활주로 6개의 기상자료로 이 심층 신경망을 통해 학습하고, 미래의 윈드시어 발생을 예측하는 실험을 진행하였다. 이 심층 신경망을 이용하여 학습된 모델은 서포트 벡터 머신 모델에 비해 평균적으로 약 13.47%의 성능 향상을 보였다
One of the most important influences on aircraft takeoff and landing is wind-shear. Wind-shear is a term that combines wind and shear. It refers to the sudden change of wind direction and velocity within a short horizontal and vertical distance. At low altitudes during take-off and landing, there is no time and space to cope with the pilots, so wind-shear can create a very dangerous situation. In this study, we propose a model for pre-predicting the wind-shear warning after a certain time using deep learning. We presented the models using deep neural networks and implemented them as an open source library, TensorFlow. We trained the deep neural networks with weather data including elements such as wind, temperature, and humidity at six runways in Incheon-airport collected by airborne observation equipment, and we predicted future wind-shear alerts. On the average, the learned model using the neural network showed about 13.47% performance improvement over the support vector machine model.
1. 서론
2. 심층 신경망
3. 실험 설계 및 결과
4. 결론 및 향후 연구