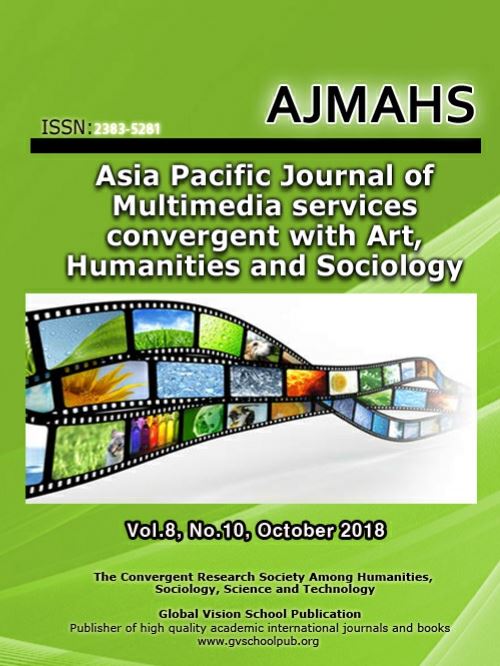
UNB 2012 침입탐지 데이터셋 기반의 네트워크 비정상 행위 탐지
Network Anomaly Detection Based on UNB 2012 Intrusion Detection Dataset
- 인문사회과학기술융합학회
- 예술인문사회융합멀티미디어논문지
- 8권 10호
- 2018.10
- 33 - 42 (10 pages)
UNB 2012 침입탐지 평가 데이터셋을 캐나다 뉴 브런스윅 대학교의 CIC (Canadian Institute for Cybersecurity)로부터 제공받았다. UNB 2012 침입탐지 데이터셋은 실제 네트워크 공격 상황을 시뮬레이션하여 생성되었다. 침입탐지와 관련하여 많은 연구가 이루어지고 있지만 대부분의 연구 결과는 실제 환경에 적용하기 어려운 측면이 있는데 CIC는 이런 점을 고려하여 UNB 2012 침입탐지 평가 데이터셋을 만들었다. 본 연구에서는 정상 클래스와 4개의 공격 클래스를 사용한다. UNB 2012 데이터셋은 일자별로 나뉜 서브 데이터셋을 갖는데 서브 데이터셋 간의 특징이 조금씩 다르다. 각 서브 데이터셋의 공통된 특징들을 선택 및 가공하여 16개의 특징을 추출한다. 대표적인 데이터 마이닝 도구 중 하나인 WEKA (Waikato Environment for Knowledge Analysis)를 사용하여 데이터셋 분할, 언더샘플링, 모델링 등의 과정을 거쳐 비교 실험을 한다. 실험 결과에서 k-NN 알고리즘이 가장 우수한 성능을 나타내었다.
UNB 2012 intrusion detection evaluation dataset was provided from CIC (Canadian Institude for Cybersecurity) of new brunswick university in Canada. The dataset is created by simulation considering real network intrusion. Many researches related to intrusion detection are conducted, but it is difficult to apply most of the results of the researches to real intrusion detection system. The CIC made the UNB 2012 intrusion detection evaluation dataset considering the difficulty. In this paper, we use a normal class and four attack classes. The dataset has several sub dataset separated by date. Each sub dataset has their own characteristics. We select or modify 16 features among common features of all sub dataset. We conduct comparative experiments after data partitioning, undersampling, and modeling using WEKA(Waikato Environment for Knowledge Analysis), a typical data mining tool. In the experimental results, the k-NN algorithm showed the best performance.
1. 서론
2. 관련연구
3. UNB 2012 데이터셋 및 실험 계획
4. 실험 결과 및 분석
5. 결론 및 향후 연구 계획