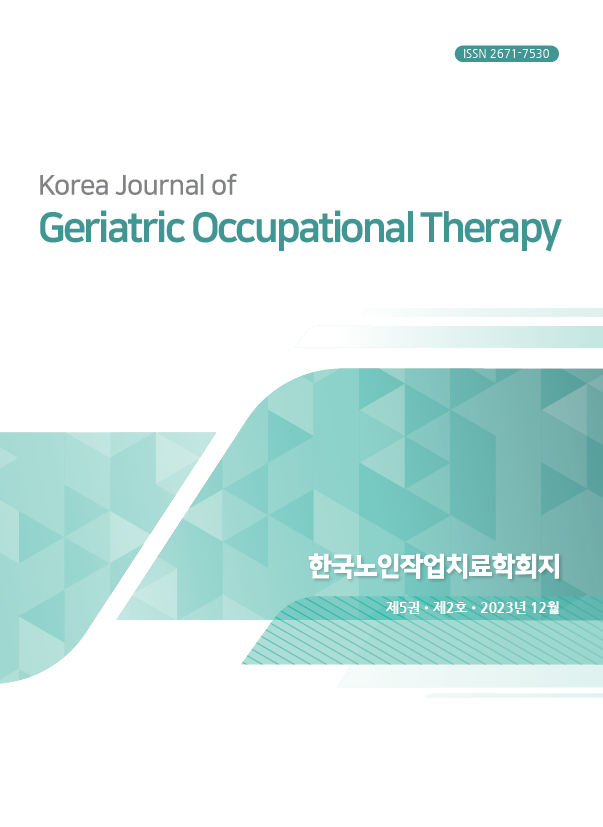
노인의 허약에 영향을 미치는 라이프스타일 요인 탐색: 의사결정나무와 랜덤 포레스트 기법을 활용하여
Exploring Lifestyle Factors Affecting Frailty Among Older Adults: Using a Decision Tree and Tandom Forest Methodology
- 한국노인작업치료학회
- 한국노인작업치료학회지
- 제5권 제2호
- 2023.12
- 65 - 83 (19 pages)
목적 : 본 연구는 기계학습 기법 중에서 의사결정나무와 랜덤 포레스트를 이용하여 노인이 허약에 영향을 미치는 라이프스타일의 위험요인과 예방요인에 대해 파악하고자 한다. 연구방법 : 고령자의 허약에 영향을 미치는 라이프스타일 요인을 규명하기 위해서 고령화 연구 패널조사의 8차 자료를 활용하여 의사결정나무와 랜덤 포레스트 기법을 적용하였다. 본 연구에서는 허약의 기준을 구분하기 위해 사용된 변수 4개와 인구학적 정보를 포함한 직간접적인 라이프스타일 요인에 관한 변수 26개가 분석에 사용되었다. 결과 : 의사결정나무에서 총 26개의 변수 중 4가지 관심 변수(Mini-mental State Examination [MMSE] 점수, 친한 사람과 만나는 횟수, 성별, 우울감 정도)가 모형에 선택되었다. 랜덤 포레스트 분석에서 중요도에 따라 ‘친한 사람과 만나는 횟수’, ‘MMSE 점수’, ‘노인구강건강평가지수’, ‘주관적인 건강 수준’, ‘나이’,‘성별’, ‘여행/관광/야외체험 횟수(년)’ ‘교육 수준’이 중요한 변수로 선택되었다. 부분 의존성 분석에서 전허약 노인의 부분 의존성 분석은 비허약이 허약으로 변하는 과도기적 양상을 보여준다. 결론 : 본 연구에서 기계학습 기법을 통해 도출된 비허약, 전허약과 허약의 차이를 보이는 라이프스타일 위험요인과 예방요인에 초점을 맞춘다면 허약을 예방하는 중재와 관리에 도움을 줄 수 있을 것이다.
Objective : This study aims to identify the risk and preventive lifestyle factors in which healthy older adults can affect frailty by using machine learning ― notably, decision trees and random forests. Methods : We used the 2020 Korean Longitudinal Study of Aging data to identify lifestyle factors affecting older adults' frailty, applying decision trees and random forest analysis. Four variables were used to distinguish the criteria for frailty, along with 26 variables related to lifestyle factors ― including demographic information. Results : Of a total of 26 variables in the decision tree, four variables (Mini-mental State Examination scores, frequency of meeting with people, sex, and level of depression) were selected as the model. In the random forest analysis, “frequency of meeting with people” the “Mini-mental State Examination score,” the “Geriatric Oral Health Assessment Index,” “subjective health status,” “age,” “sex,” “the number of travel/tourism/outdoor experiences,” and “education level” were selected as important variables. In the partial dependence analysis, pre-frailty displayed a transitional pattern of non-frailty, turning into frailty. Conclusion : Focusing on factors that show the difference among non-frailty, pre-frailty, and frailty derived from this study can help in the intervention and management of preventing frailty by using a machine learning technique.
Ⅰ. 서론
Ⅱ. 연구 방법
Ⅲ. 연구 결과
Ⅳ. 고찰
Ⅴ. 결론